Introduction to Image Recognition
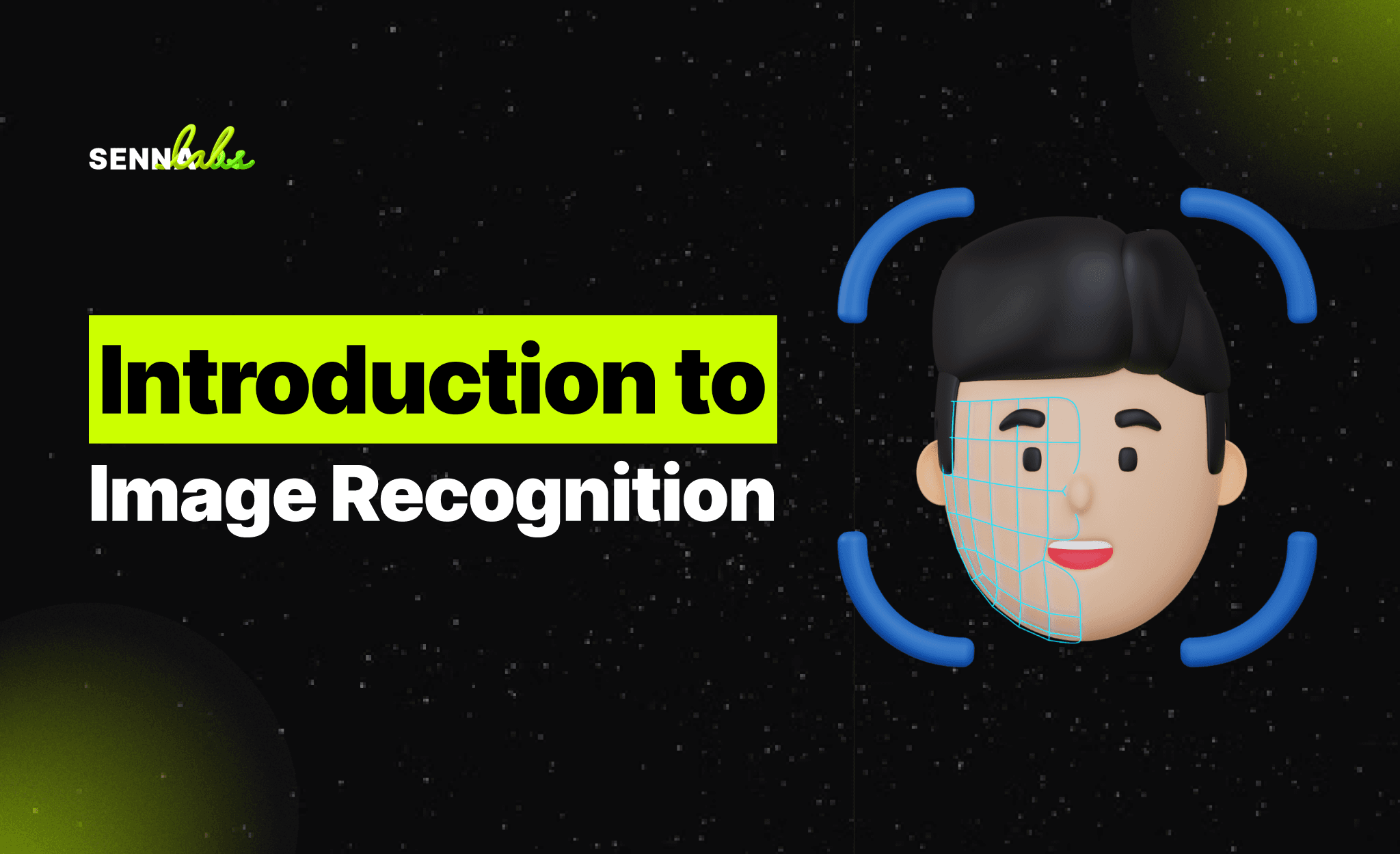
Image recognition is the processing of the image seen by the machine (computer) in such a way that by analyzing the digital data recording. It is possible to classify the observed objects in order to make further decisions.
Image recognition in the meaning of e.g. recognizing people on photos is actually a special case of the pattern recognition domain that applies in many areas of science. Image recognition is one of the oldest research areas in computing. Most people think of large-scale projects when they hear the term, such as engines that can identify broad categories of objects.
The ability to distinguish between a dog on a lawn and a cat on a couch and then to correctly label the animal and setting is the capability of image recognition today. Applying such labels to an image is called “image classification.” Algorithms written by companies like Google and academic universities like Stanford have pushed image recognition to this point.
Many use cases need more powerful or more precise feedback from computers than simple classification. Companies use video analytics, for example, to identify the movement of human-sized objects, a relatively easy task when you know where the camera is positioned and looking.
Four main computer vision tasks used today in real-world applications
-
Classification.
-
Tagging.
-
Detection.
-
Segmentation.
Four main/ basic types in image classification
General object detection framework
1. Region proposals
Originally, the ‘selective search’ algorithm was used to generate object proposals. Lillie Weng provides a thorough explanation of this algorithm in her blog post. In short, a selective search is a clustering-based approach that attempts to group pixels and generate proposals based on the generated clusters.
An example of a selective search
An important trade-off that is made with region proposal generation is the number of regions vs. the computational complexity. The more regions you generate, the more likely you will be able to find the object. On the flip-side, if you exhaustively generate all possible proposals, it won’t be possible to run the object detector in real-time.
2. Feature extraction
The goal of feature extraction is to reduce a variable-sized image to a fixed set of visual features. Image classification models are typically constructed using strong visual feature extraction methods. Whether they are based on traditional computer vision approaches, such as for example, filter based approached, histogram methods, etc., or deep learning methods, they all have the exact same objective: extract features from the input image that are representative for the task at hands and use these features to determine the class of the image.
Feature extraction from region proposal scheme
3. Evaluation metric
The most common evaluation metric that is used in object recognition tasks is ‘mAP’, which stands for ‘mean average precision’. It is a number from 0 to 100 and higher values are typically better, but its value is different from the accuracy metric in classification.
Pixel Accuracy
An alternative metric to evaluate a semantic segmentation is to simply report the percent of pixels in the image which were correctly classified. The pixel accuracy is commonly reported for each class separately as well as globally across all classes.
To evaluate our collection of predicted masks, we'll compare each of our predicted masks with each of the available target masks for a given input.
-
A true positive is observed when a prediction-target mask pair has an IoU score that exceeds some predefined threshold.
-
A false positive indicates a predicted object mask had no associated ground truth object mask.
-
A false negative indicates a ground truth object mask had no associated predicted object mask.
This is the end of this article for introducing image recognition. In the next article, we will demonstrate how to implement image recognition from zero!!
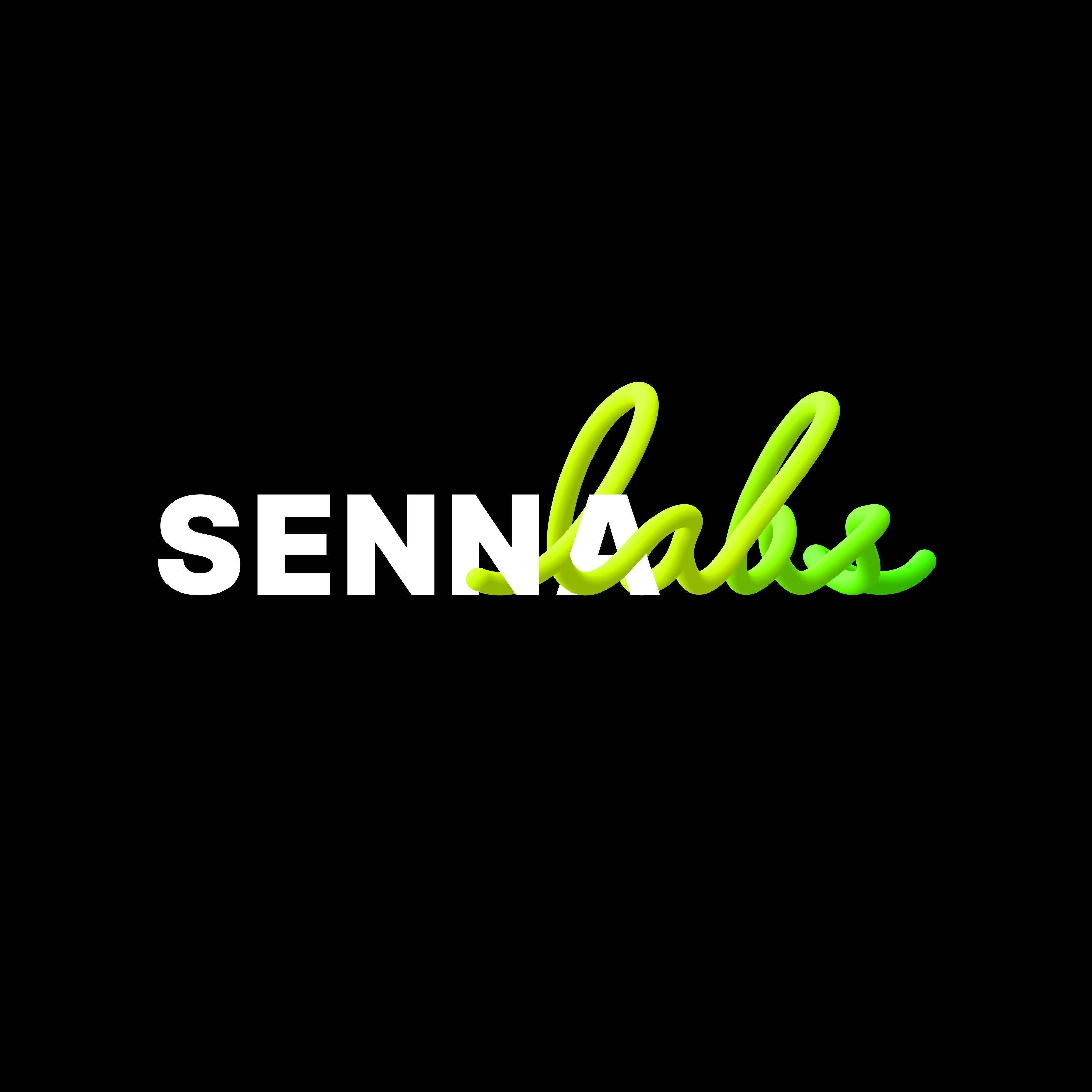
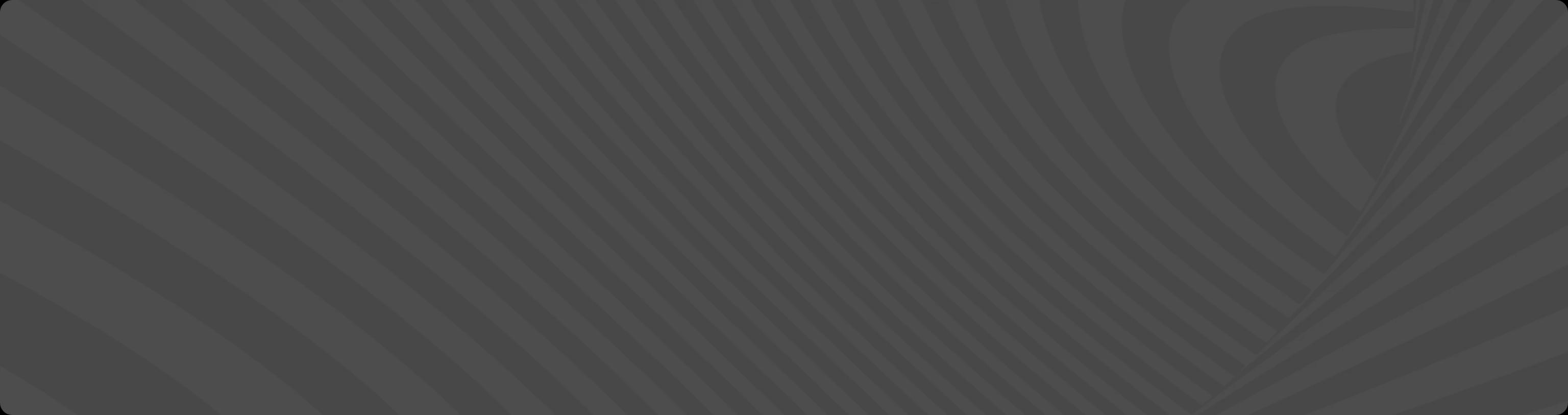
Subscribe to follow product news, latest in technology, solutions, and updates
บทความอื่นๆ
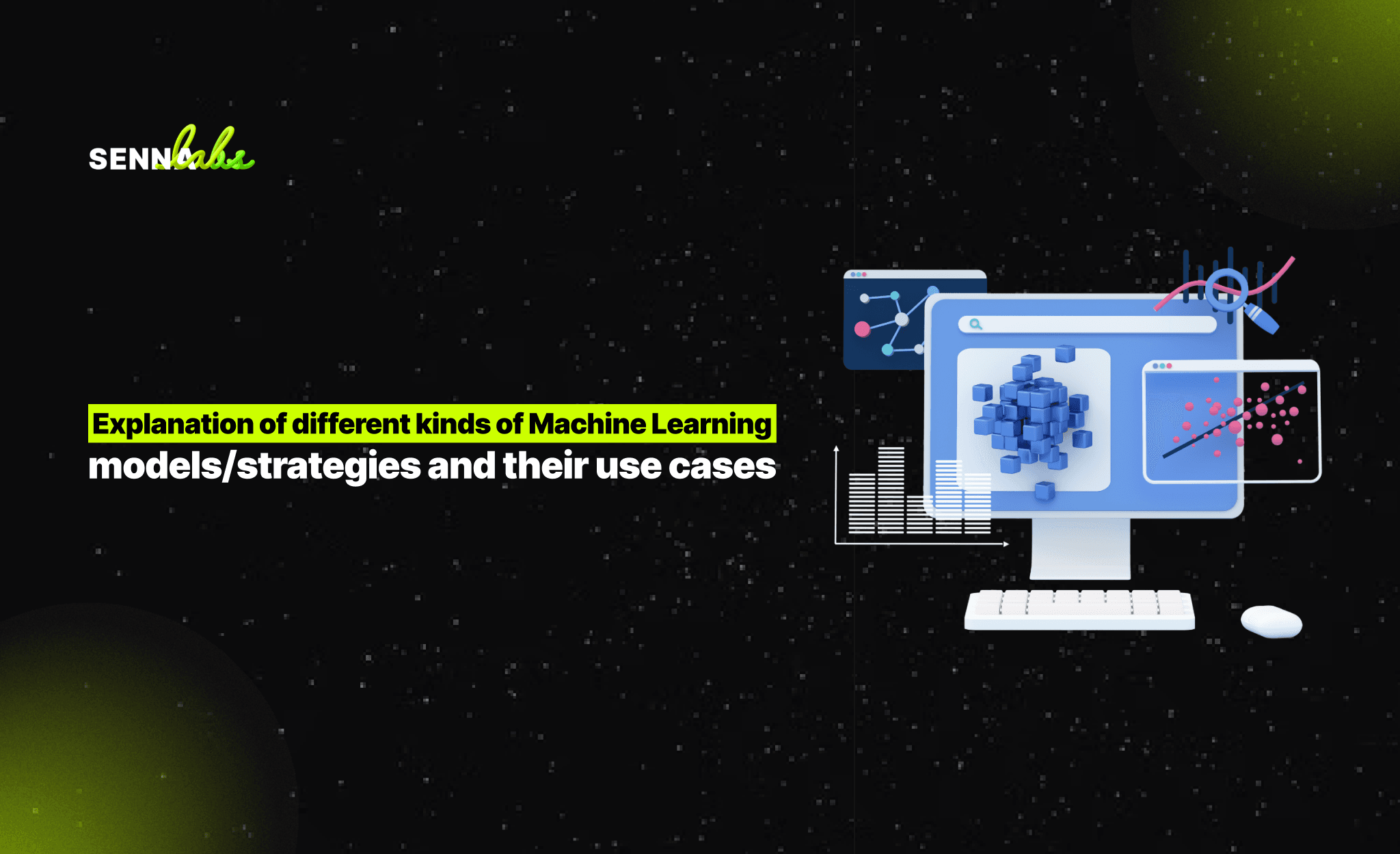
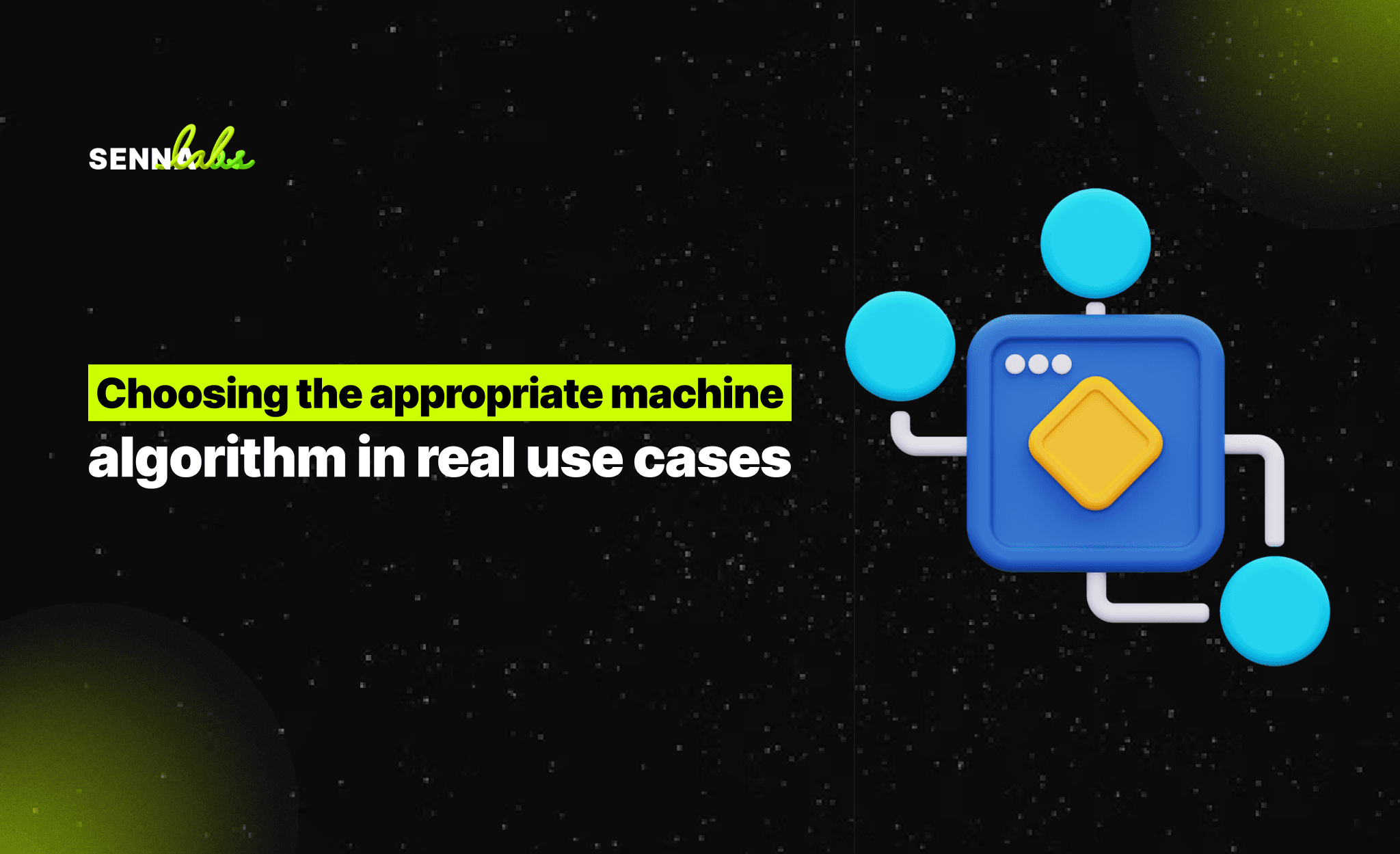
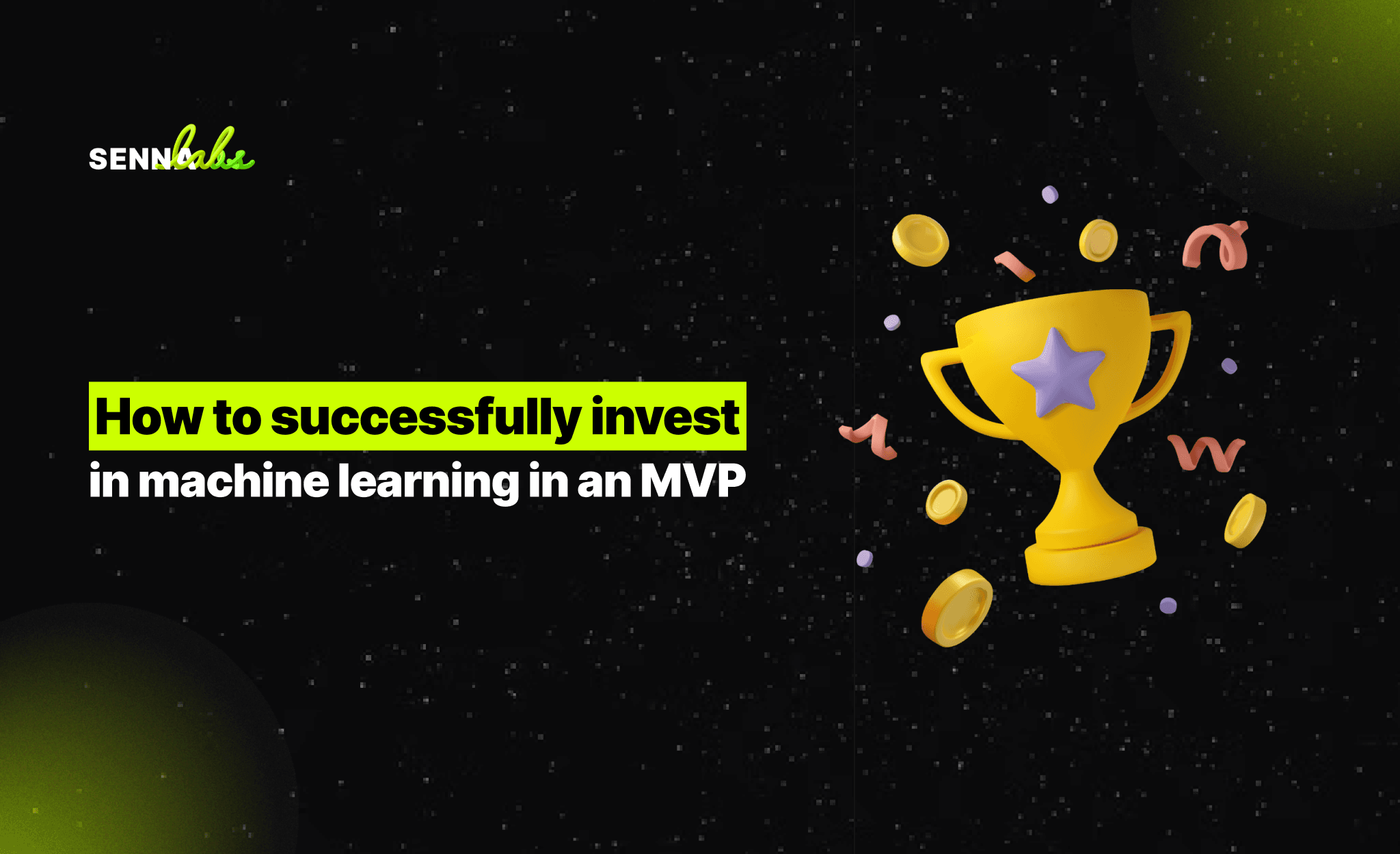
Let’s build digital products that are simply awesome !
We will get back to you within 24 hours!ติดต่อเรา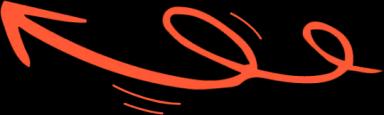
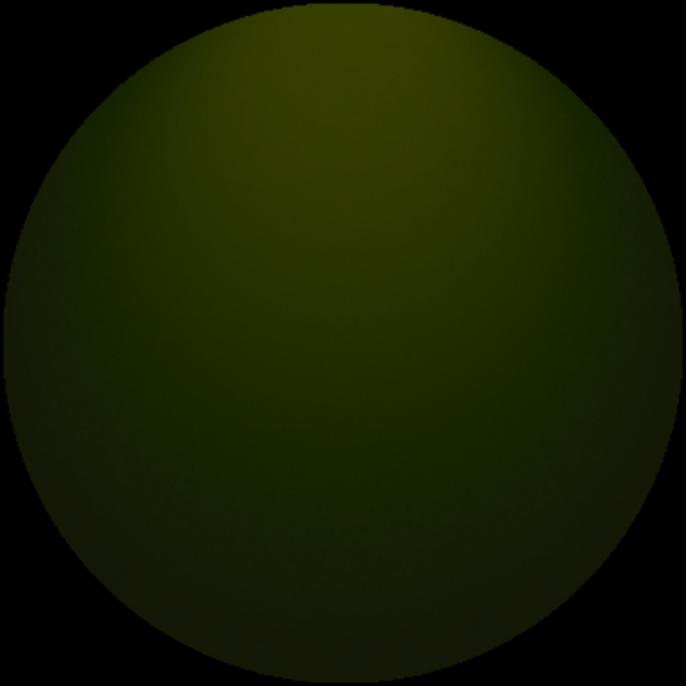
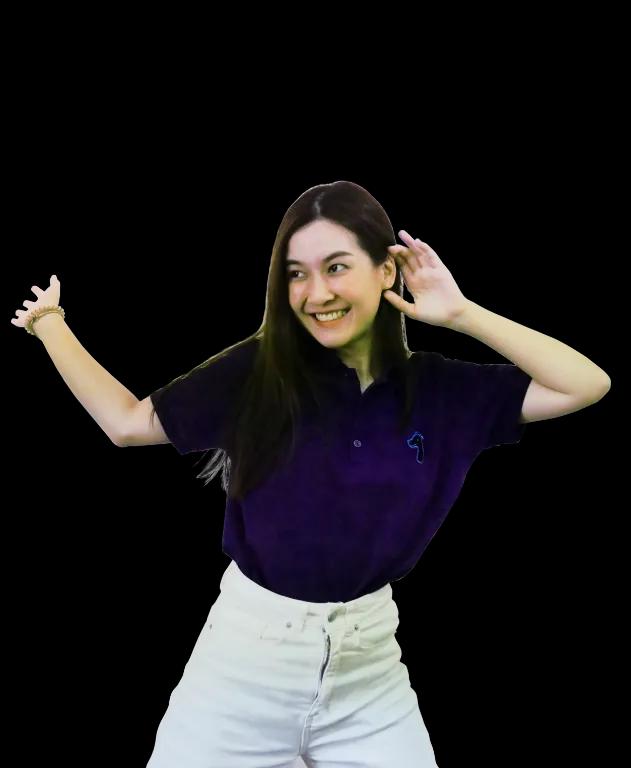
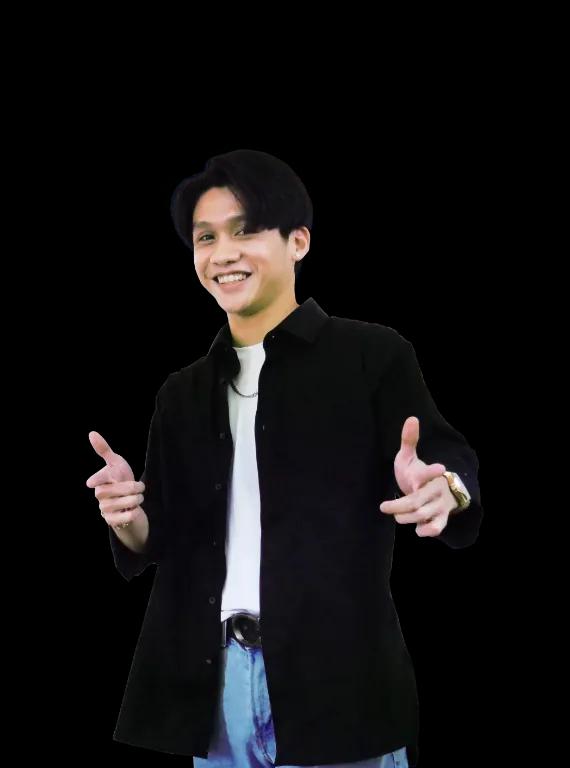
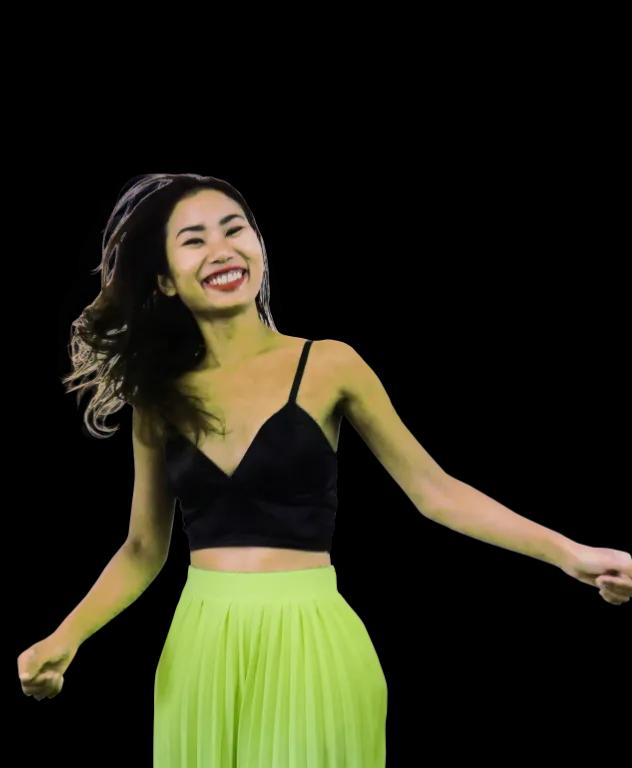
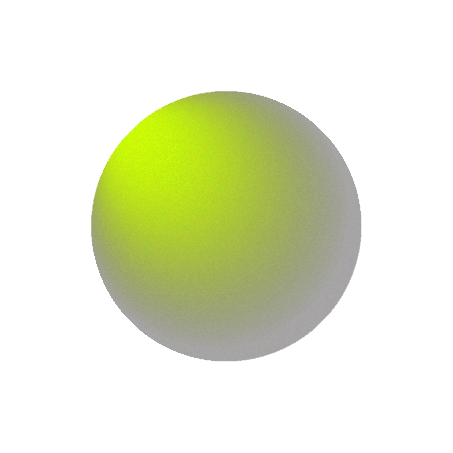
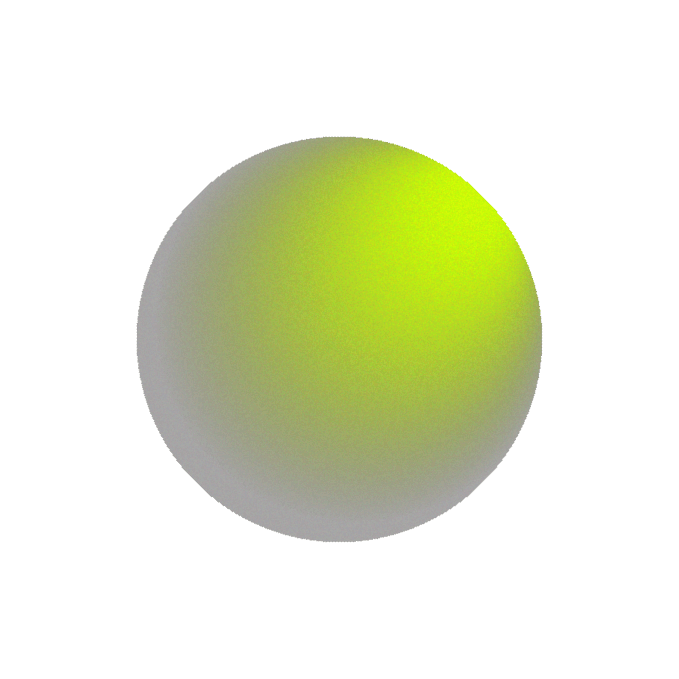
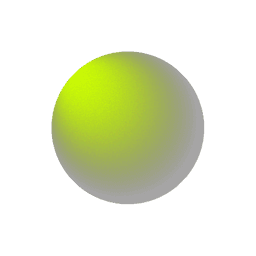
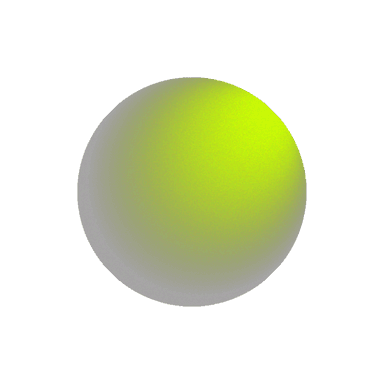
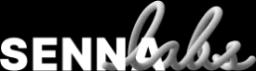