How to successfully invest in machine learning in an MVP
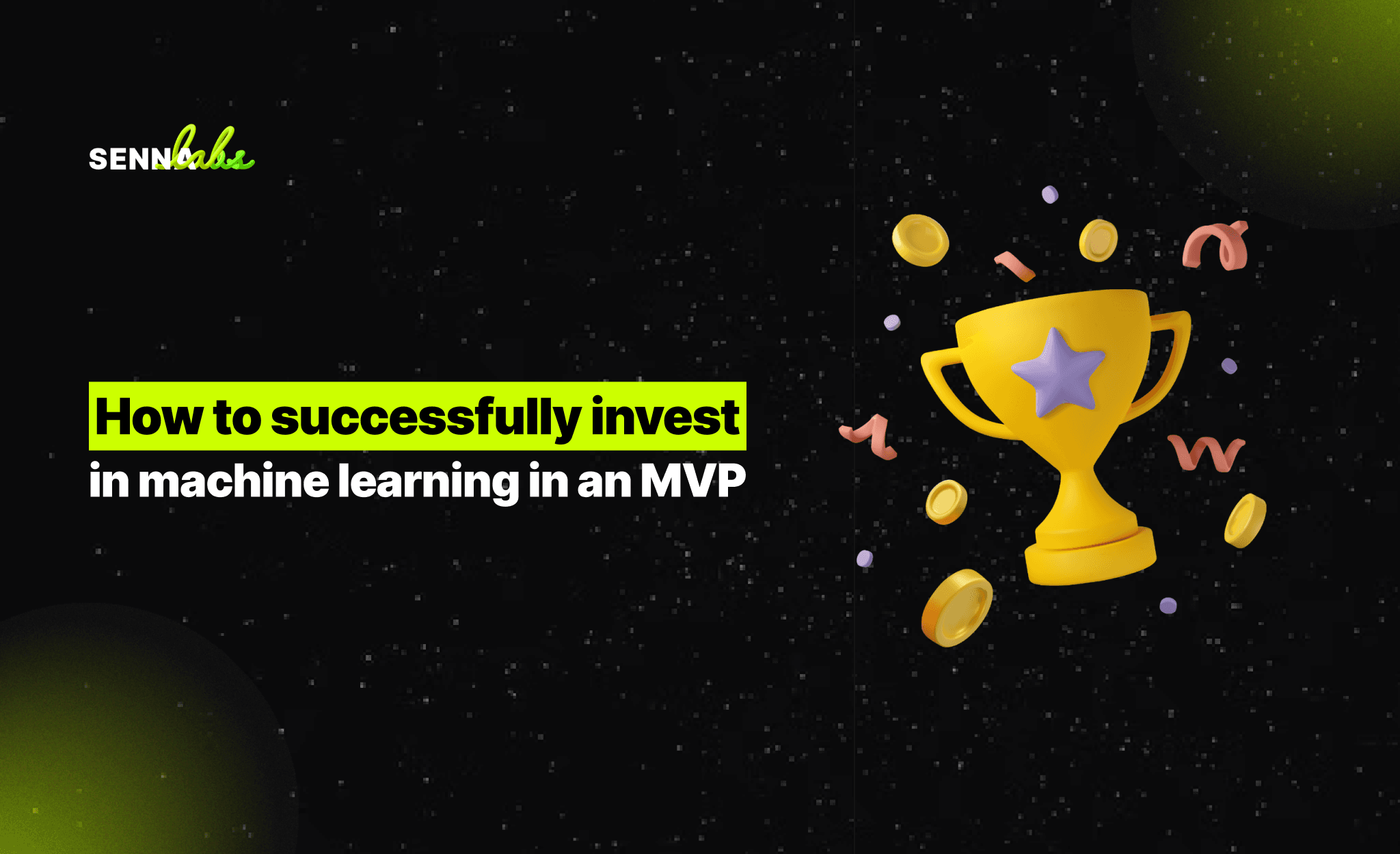
A minimum viable product (MVP) is a version of a product with contains enough features to satisfy early customers and validate ideas early in the development cycle for future development. The MVP can help the product team receive user feedback to iterate and improve product development.
"The version of a new product which allows a team to collect the maximum amount of validated learning about customers with the best effort"
Eric Ries, defined an MVP
In the traditional software development cycle, MVPs are a common part of the lean development cycle. There are tons of ways to explore or study a market to learn about the challenges related to the product before development. While machine learning product development is quite difficult to become a lean discipline because it's hard to learn and make reliably from complex systems.
For the machine learning product, building MVPs is absolutely necessary. If the weakness in the model originates from bad data quality, all further investments to improve the model will be doomed to failure, no matter the amount of money thrown at the project. Similarly, if the model underperforms because it was not deployed or monitored properly, then any money spent on improving data quality will be wasted. Teams can avoid these pitfalls by first developing an MVP and by learning from failed attempts. Before you launch your machine learning model, start with an MVP - Jennifer Prendki
Machine learning investment strategy
Generally, machine learning product development requires huge overhead work, Ex. the design of the machine learning model pipeline, dataset preparation, and cleaning process, which almost of machine learning develops product stuck on this step or data management frameworks and data visualization systems. This kind of works causes an 'S' shaped return-investment curve. Which if company leaders don't properly manage this S-shaped ROI, the project might be failure.
The return-on-investment curve of Machine Learning initiatives compared to traditional software development projects.
Machine learning investment for building an MVP
- Data collection: the costs are vary based on the type of product you are building and how often you are gathering and updating data.
- Data pipeline building: the data transferring pipeline is a one-time initiative, however, it is also costly and time-consuming.
- Data storage: storage will become extremely expensive and will require that we stick to the bare minimum: only the data that is truly informational and actionable.
- Data cleaning: the process is all the more costly since the amount of data always on the rise when data science will take in charge of developing the model.
- Data annotation: naturally, large amounts of data requires more label, and using crowds of human annotators isn’t enough anymore. Semi-automated labeling and active learning are becoming increasingly attractive to many companies, especially those with very large volumes of data. However, the licenses to those platforms can represent a substantial addition to the entire price of your ML initiative.
- Compute power: The machine learning training process required a huge amount of mathematical processing units, with large volumes of data and complex models, the bill can become a considerable part of the entire budget and can sometimes even require a hefty investment in a server solution.
- Modeling cost: The model development phase accounts for the most unpredictable cost in your final bill because the amount of time required to build a model depends on many different factors: the skill of your ML team, problem complexity, required accuracy, data quality, time constraints, and even luck. Hyperparameter tuning for deep learning is making things even more hectic, as this phase of development benefits little from experience, and usually, only a trial-and-error approach prevails. - Anastasia Kryhanovska
- Deployment cost: this phase might cause the most time-consuming and expensive part of creating machine learning MVPs depends on your project size
How to succeed with an ML's MVP
-
Data scientists need to evaluate the data and the model separately
Data sciences must keep this fact in mind since, they now have the option of improving their data collecting process, they can do justice to those models that would have been otherwise identified as hopeless.
-
Be patient with ROI
S-shaped, even MVPs require more way to work than you could typically anticipate. As we have seen, ML products require many complex steps to reach completion, and this is something that needs to be profusely communicated to stakeholders to limit the risk of frustration and premature abandonment of a project.
-
Diagnosing is costly but critical
Debugging ML systems is almost always extremely time-consuming, in particular, because of the lack of explainability in many modern models (such as Deep Learning). Diagnosing problems also gives your team the opportunity to learn valuable lessons from their mistakes, potentially shortening future project cycles.
Make It happen
This article makes you aware that, developing machine learning MVPs is not that easy and there is no shortcut, and no matter how long your team experienced working in the AI field but ML models are extremely powerful than you thought especially when the data is highly dimensional and high volume. You need to test, prove your models very early in the MVP phase and invest the time and money to fixing to weaknesses. Next article we look up closer to build the machine learning model and see how's powerful.
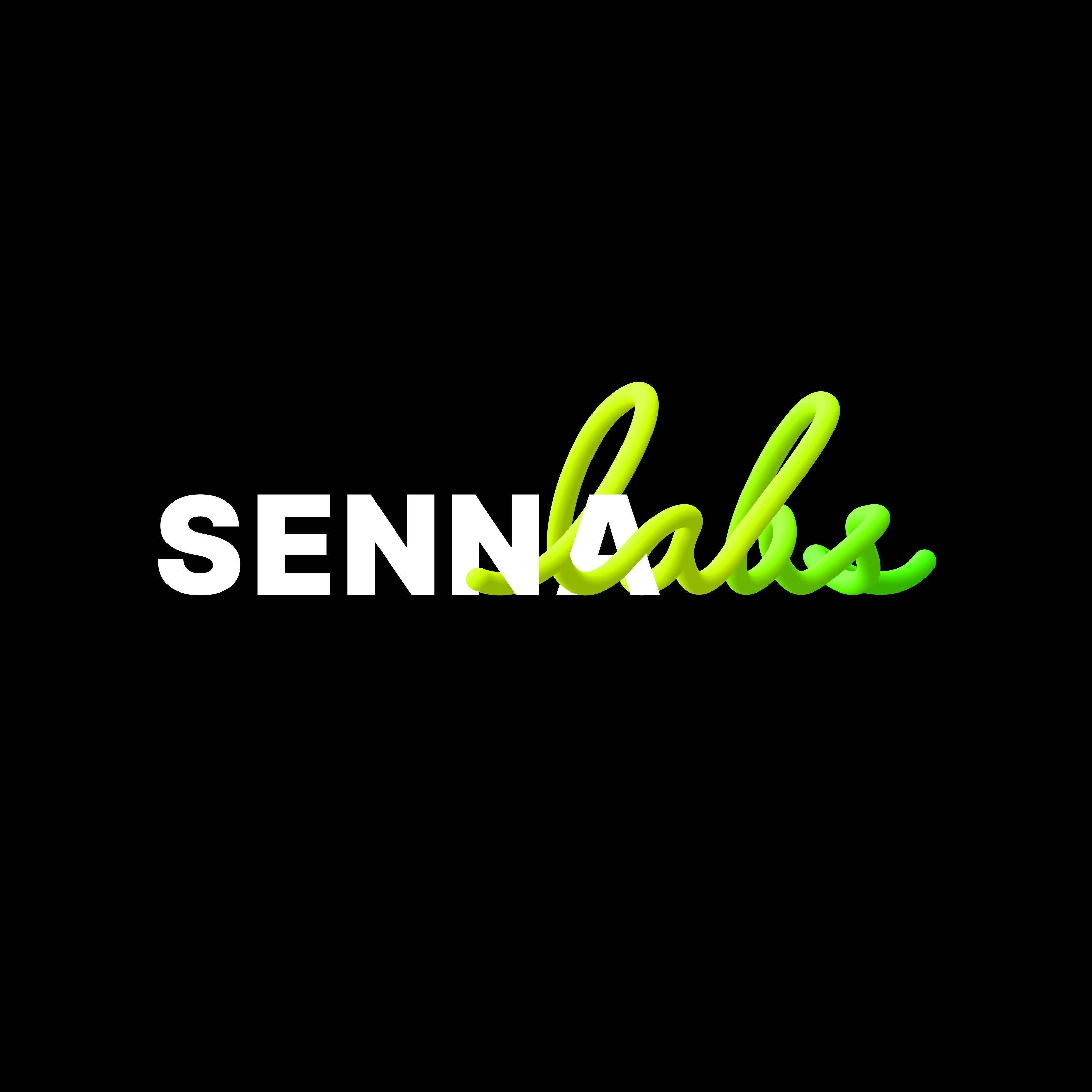
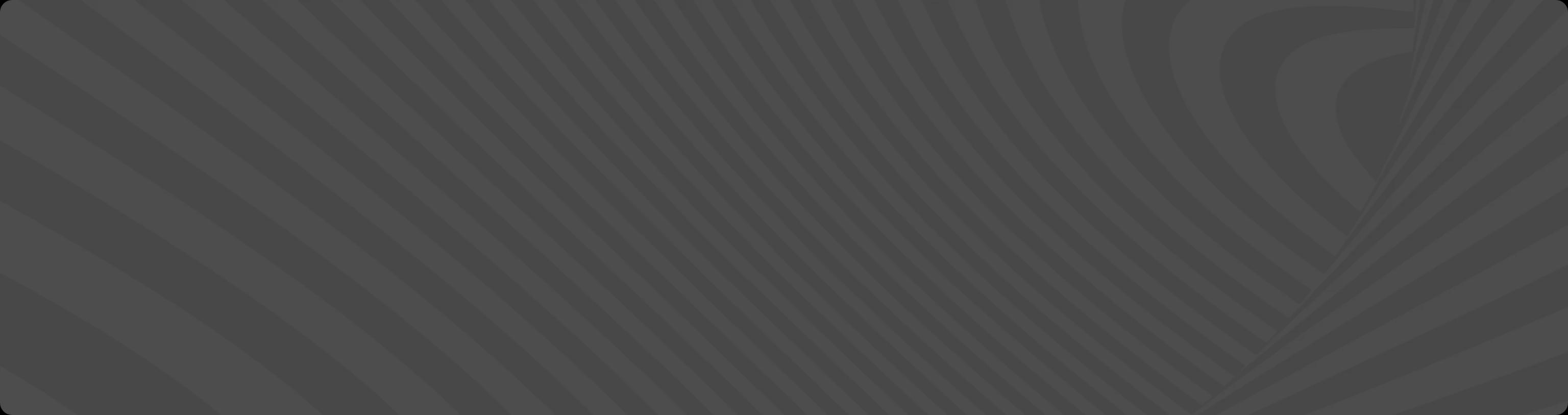
Subscribe to follow product news, latest in technology, solutions, and updates
บทความอื่นๆ
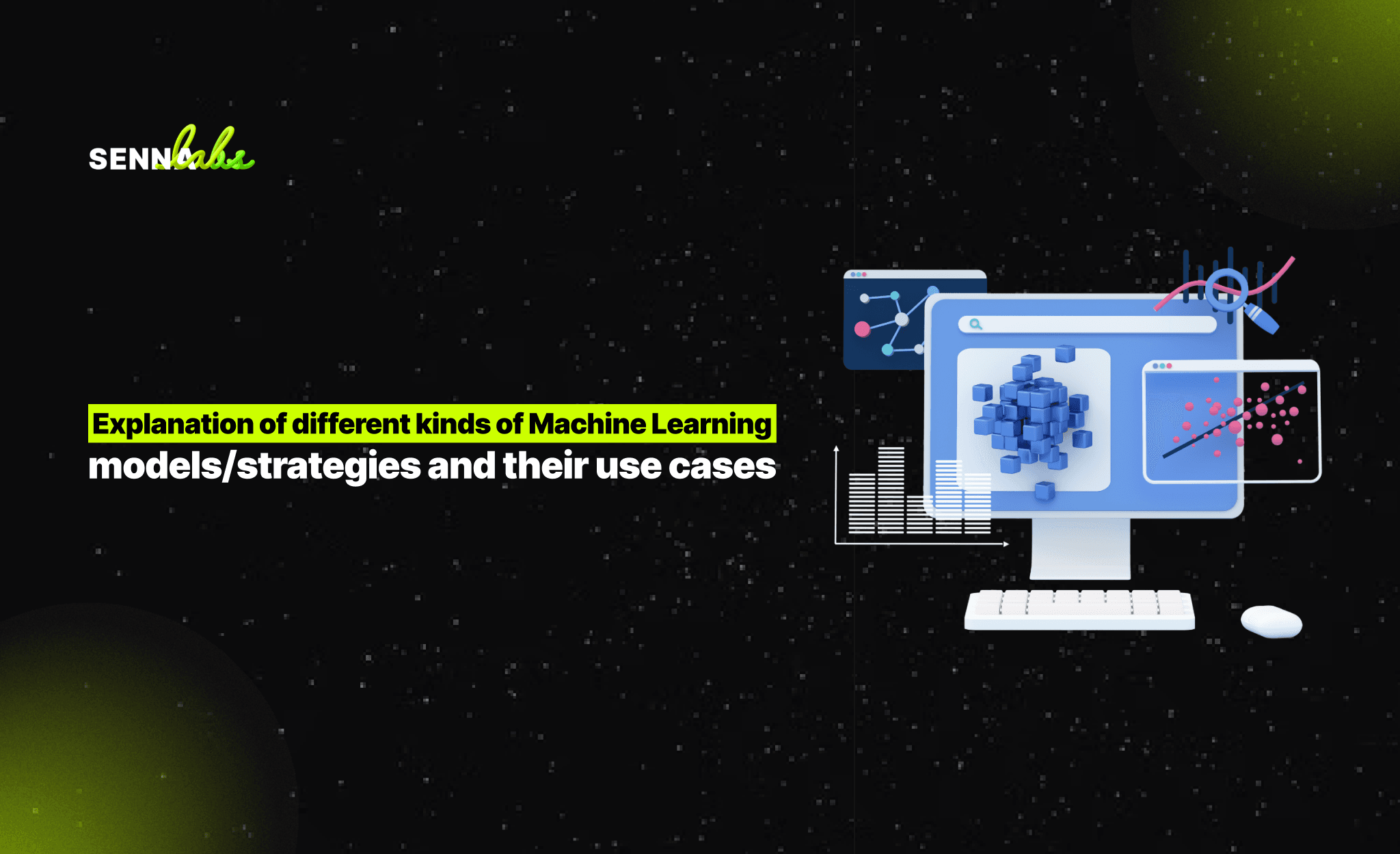
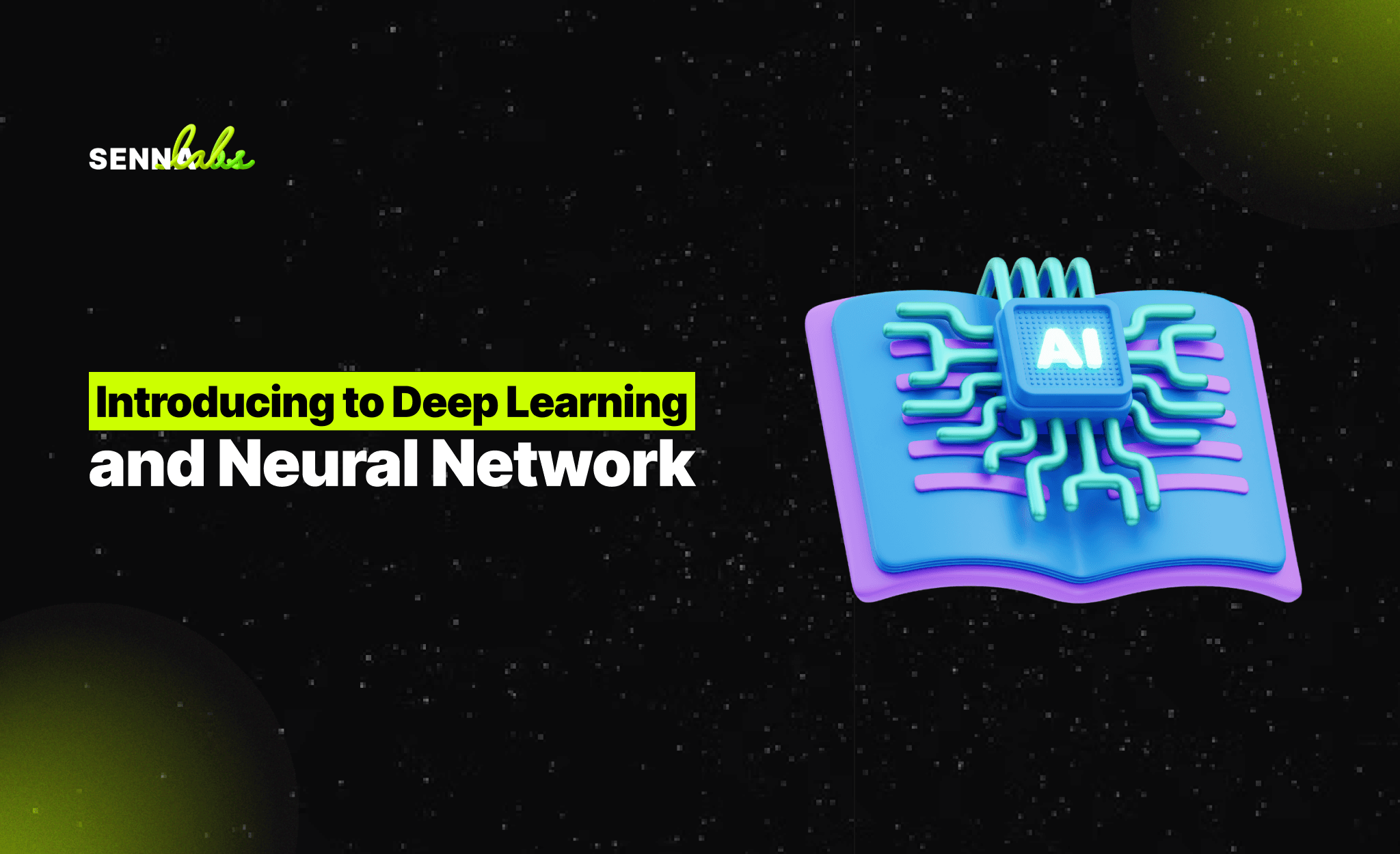
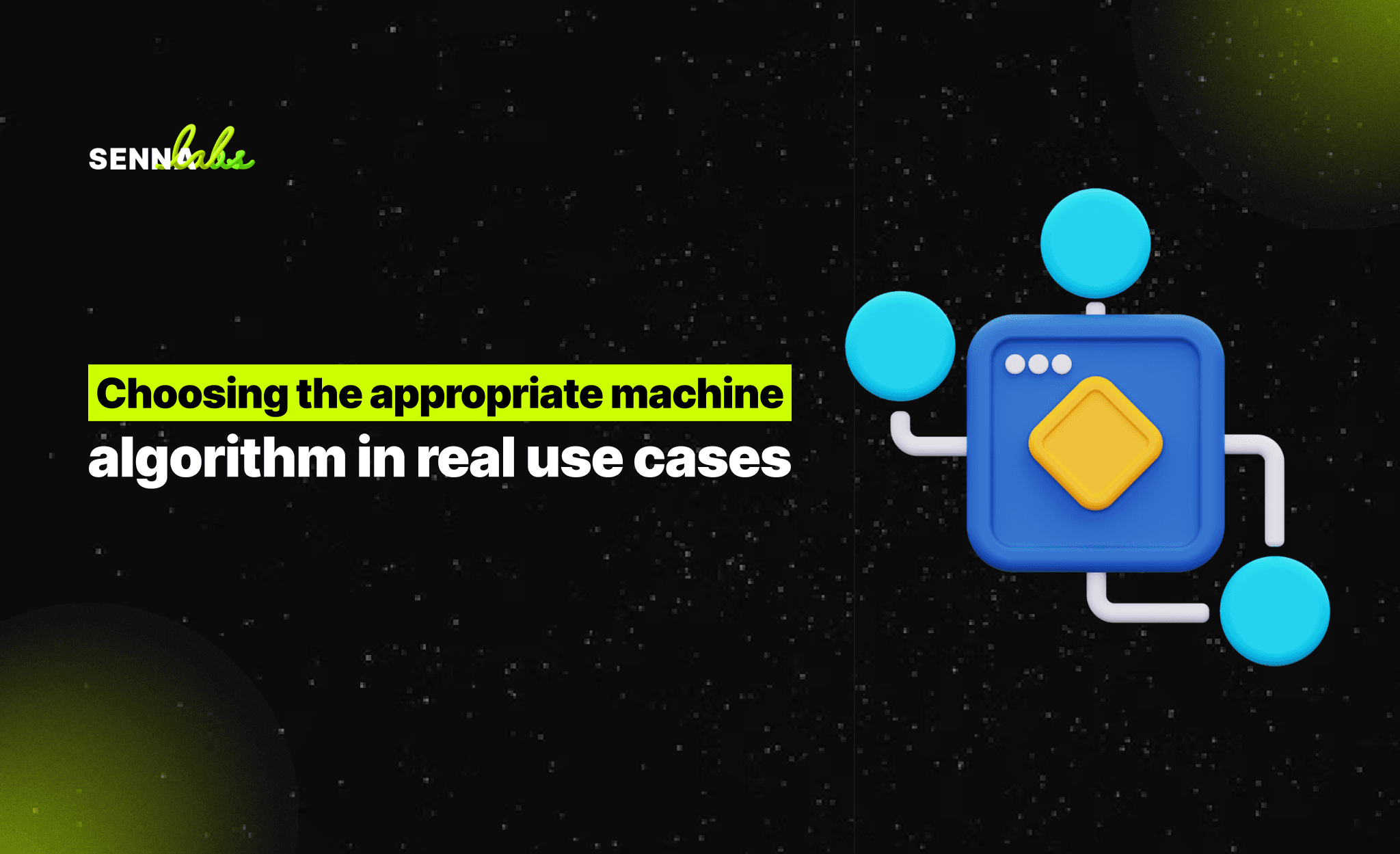
Let’s build digital products that are simply awesome !
We will get back to you within 24 hours!ติดต่อเรา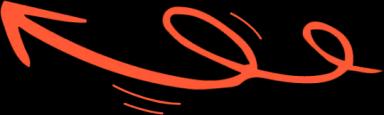
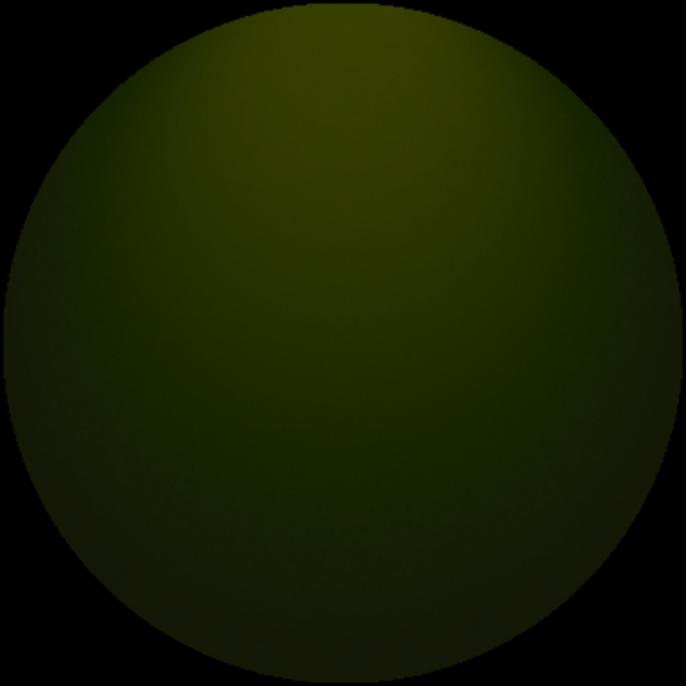
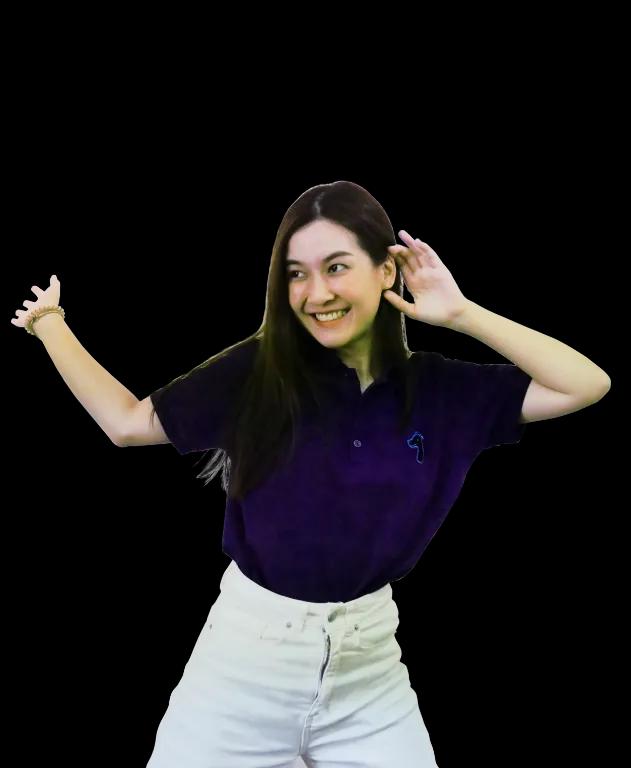
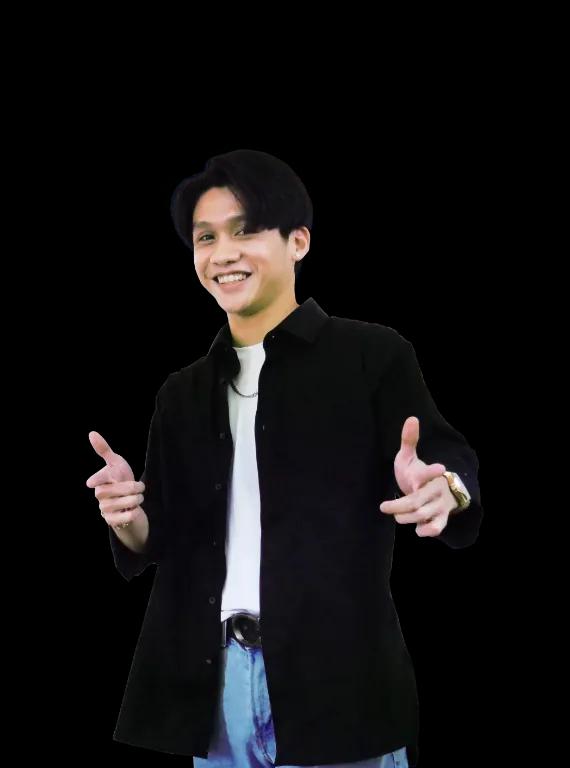
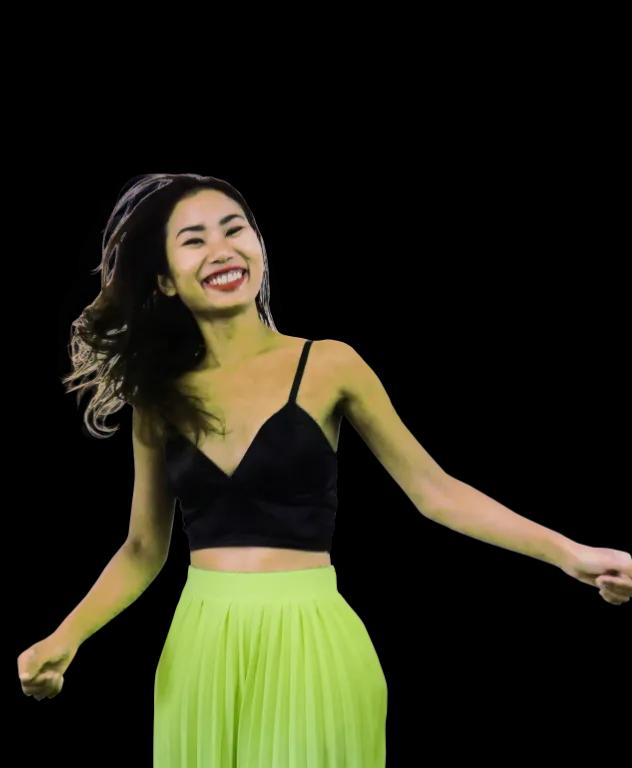
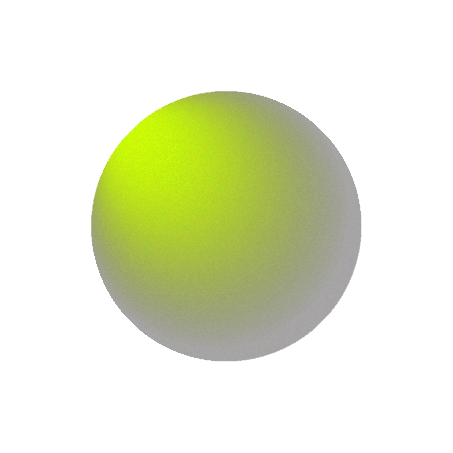
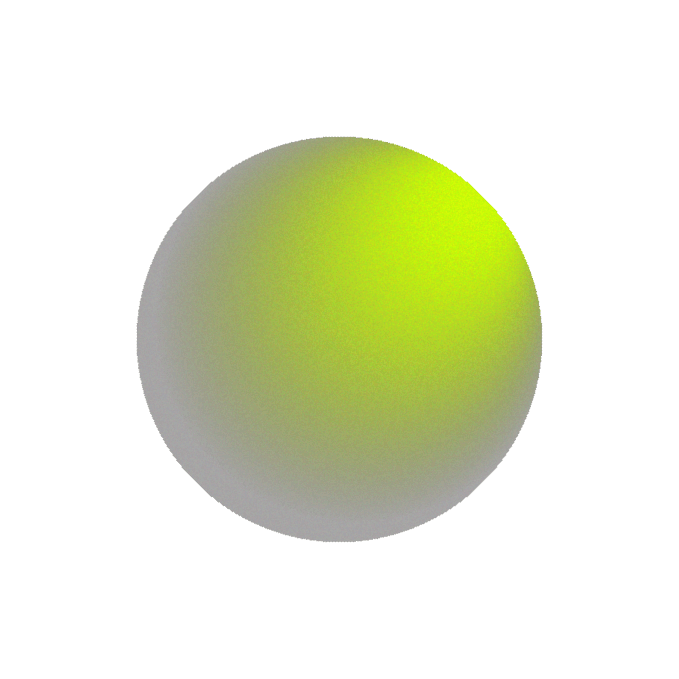
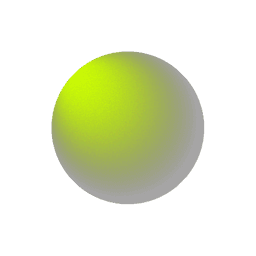
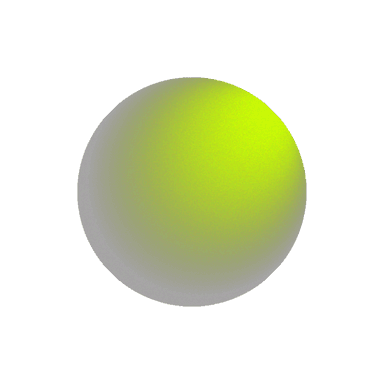
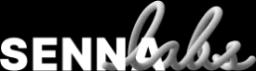