Optimizing Manufacturing with AI Agents: Predictive Maintenance
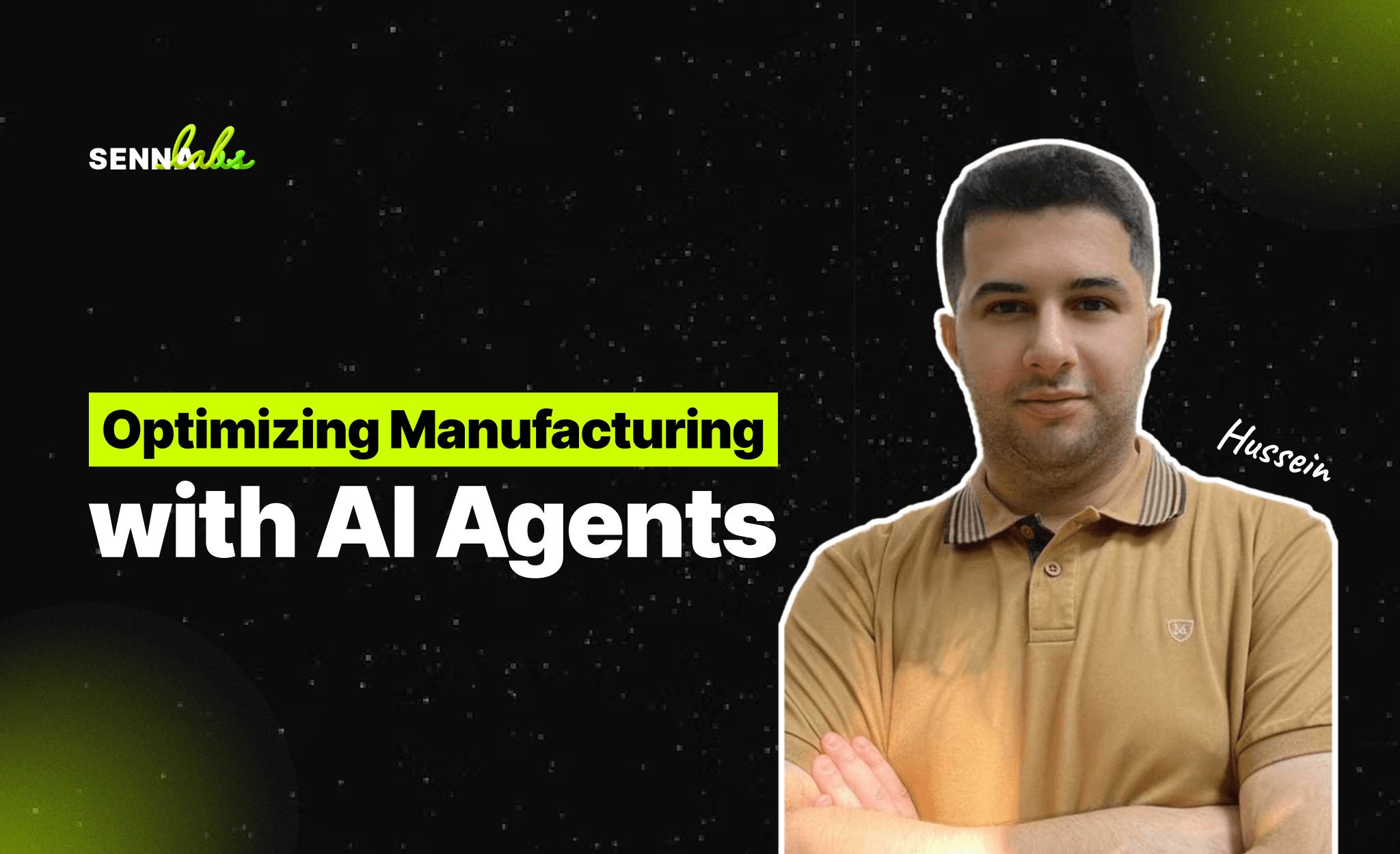
In the manufacturing industry, unplanned downtime and equipment failures can lead to significant productivity losses and increased costs. Artificial Intelligence (AI) is transforming manufacturing operations by introducing predictive maintenance, a proactive approach to equipment management. AI systems monitor machinery performance in real-time, analyze patterns, and predict potential failures, enabling timely intervention to minimize disruptions.
This article explores how AI agents optimize manufacturing processes through predictive maintenance, focusing on the benefits, implementation strategies, and best practices.
The Need for Predictive Maintenance
Traditional maintenance methods, such as reactive and scheduled maintenance, often fall short in optimizing equipment performance:
-
Reactive Maintenance: Repairs are made after equipment fails, leading to unexpected downtime.
-
Scheduled Maintenance: Preventive tasks are performed at fixed intervals, often resulting in unnecessary downtime or missed issues.
Predictive maintenance powered by AI addresses these challenges by using real-time data to predict failures and optimize maintenance schedules.
How AI Agents Enable Predictive Maintenance
AI agents leverage advanced technologies like machine learning, IoT sensors, and big data analytics to monitor and predict equipment performance:
1. Real-Time Monitoring
AI systems collect data from IoT sensors embedded in machinery, tracking key performance metrics such as:
-
Temperature
-
Vibration
-
Pressure
-
Energy consumption
Example: A factory monitors the vibration levels of a conveyor belt motor to detect signs of wear or misalignment.
2. Anomaly Detection
Machine learning models analyze historical and real-time data to identify deviations from normal operating conditions.
-
AI alerts operators when anomalies suggest potential issues, such as overheating or unusual noise.
Example: An AI agent detects abnormal vibration patterns in a lathe machine, signaling potential bearing failure.
3. Failure Prediction
AI systems use predictive analytics to estimate the time-to-failure of critical components, enabling proactive maintenance.
-
Predictive models consider factors like usage history, environmental conditions, and material fatigue.
Example: AI predicts that a hydraulic pump in a production line will fail within two weeks based on performance trends.
4. Maintenance Optimization
AI agents recommend optimal maintenance schedules, ensuring repairs are made only when necessary to prevent downtime.
-
Dynamic scheduling reduces unnecessary maintenance tasks and maximizes equipment lifespan.
Example: A factory reschedules maintenance for a robotic arm based on AI recommendations, aligning with low-production periods.
Benefits of Predictive Maintenance with AI
-
Reduced Downtime: By addressing issues before they escalate, AI minimizes unplanned equipment failures.
-
Cost Savings: Proactive maintenance reduces repair costs, spare part inventory, and labor expenses.
-
Extended Equipment Lifespan: Timely interventions prevent excessive wear and tear, maximizing machinery longevity.
-
Improved Productivity: Fewer disruptions allow production schedules to run smoothly.
-
Enhanced Safety: Early detection of issues reduces the risk of accidents caused by equipment failures.
Example Outcome: A factory implementing AI-driven predictive maintenance reduced unplanned downtime by 30% and saved $500,000 annually in repair costs.
Use Case: Predictive Maintenance in Action
Problem:
A manufacturing plant experienced frequent unplanned downtime due to unforeseen equipment failures, disrupting production and increasing costs.
Solution:
The plant deployed an AI predictive maintenance system to monitor critical machinery in real-time.
Implementation:
-
Sensor Installation: IoT sensors were installed on key equipment to track performance metrics.
-
Data Collection: Historical and real-time data were integrated into the AI system.
-
AI Analysis: Machine learning models analyzed the data to detect anomalies and predict failures.
-
Actionable Insights: AI provided alerts and recommended maintenance schedules based on failure predictions.
Results:
-
Unplanned downtime decreased by 40%.
-
Maintenance costs were reduced by 25%.
-
Equipment lifespan increased by 20%.
Steps to Implement Predictive Maintenance
Step 1: Identify Critical Equipment
Start by selecting machinery that significantly impacts production or frequently experiences downtime.
Step 2: Install IoT Sensors
Equip machines with sensors to collect performance data such as temperature, vibration, and energy use.
Step 3: Choose an AI Platform
Select a predictive maintenance platform with features like anomaly detection, real-time monitoring, and failure prediction.
Examples: GE’s Predix, IBM’s Maximo APM, or Siemens’ MindSphere.
Step 4: Train AI Models
Use historical data to train machine learning models, ensuring they can accurately predict potential failures.
Step 5: Monitor and Optimize
Continuously monitor AI predictions, update models with new data, and refine maintenance schedules for maximum efficiency.
Challenges and Solutions
Challenge 1: High Initial Investment
Solution: Start with a pilot project to demonstrate ROI before scaling across the entire facility.
Challenge 2: Data Quality Issues
Solution: Ensure data collection systems are robust, and clean historical data before training AI models.
Challenge 3: Resistance to Change
Solution: Provide training for staff and highlight the benefits of predictive maintenance, such as reduced workloads and improved safety.
Future of AI in Predictive Maintenance
As AI technology evolves, predictive maintenance will become even more advanced:
-
Self-Learning Systems: AI models will continuously improve without manual retraining.
-
Integration with Digital Twins: AI will simulate equipment performance in virtual environments for better predictions.
-
Edge Computing: AI will process data directly on devices, enabling faster and more efficient predictions.
-
Cross-Industry Adoption: Predictive maintenance will expand to industries beyond manufacturing, including healthcare and transportation.
Conclusion
AI-driven predictive maintenance is transforming manufacturing by reducing downtime, cutting costs, and improving operational efficiency. By leveraging real-time monitoring, anomaly detection, and failure prediction, manufacturers can proactively address equipment issues before they escalate.
For the manufacturing plant in this use case, implementing AI for predictive maintenance resulted in significant cost savings, extended equipment life, and improved productivity. As AI technology continues to evolve, predictive maintenance will become an essential tool for manufacturers seeking to stay competitive in a rapidly changing industry.

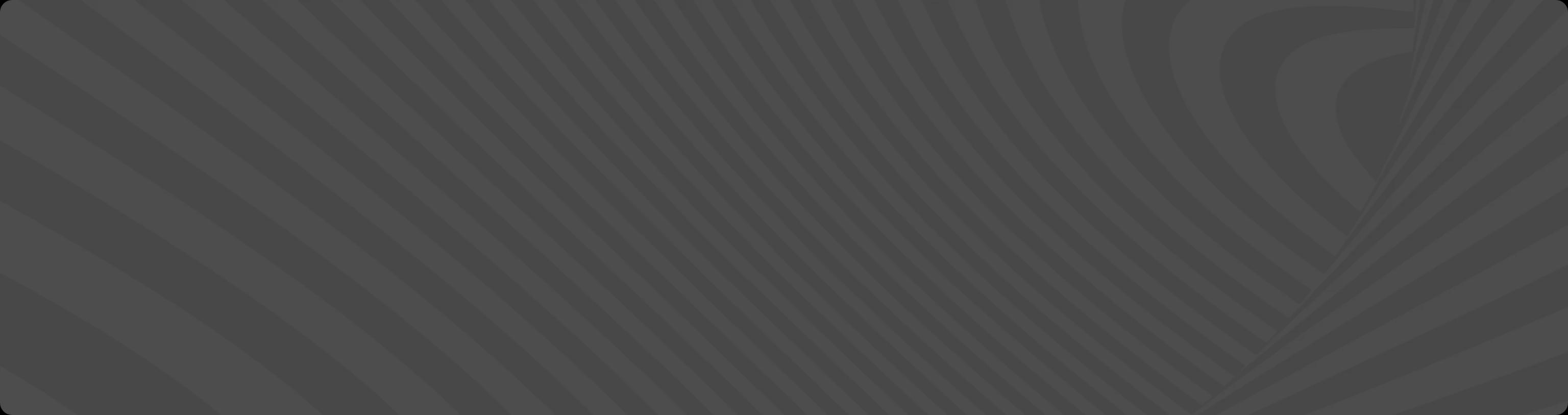
Subscribe to follow product news, latest in technology, solutions, and updates
Other articles for you
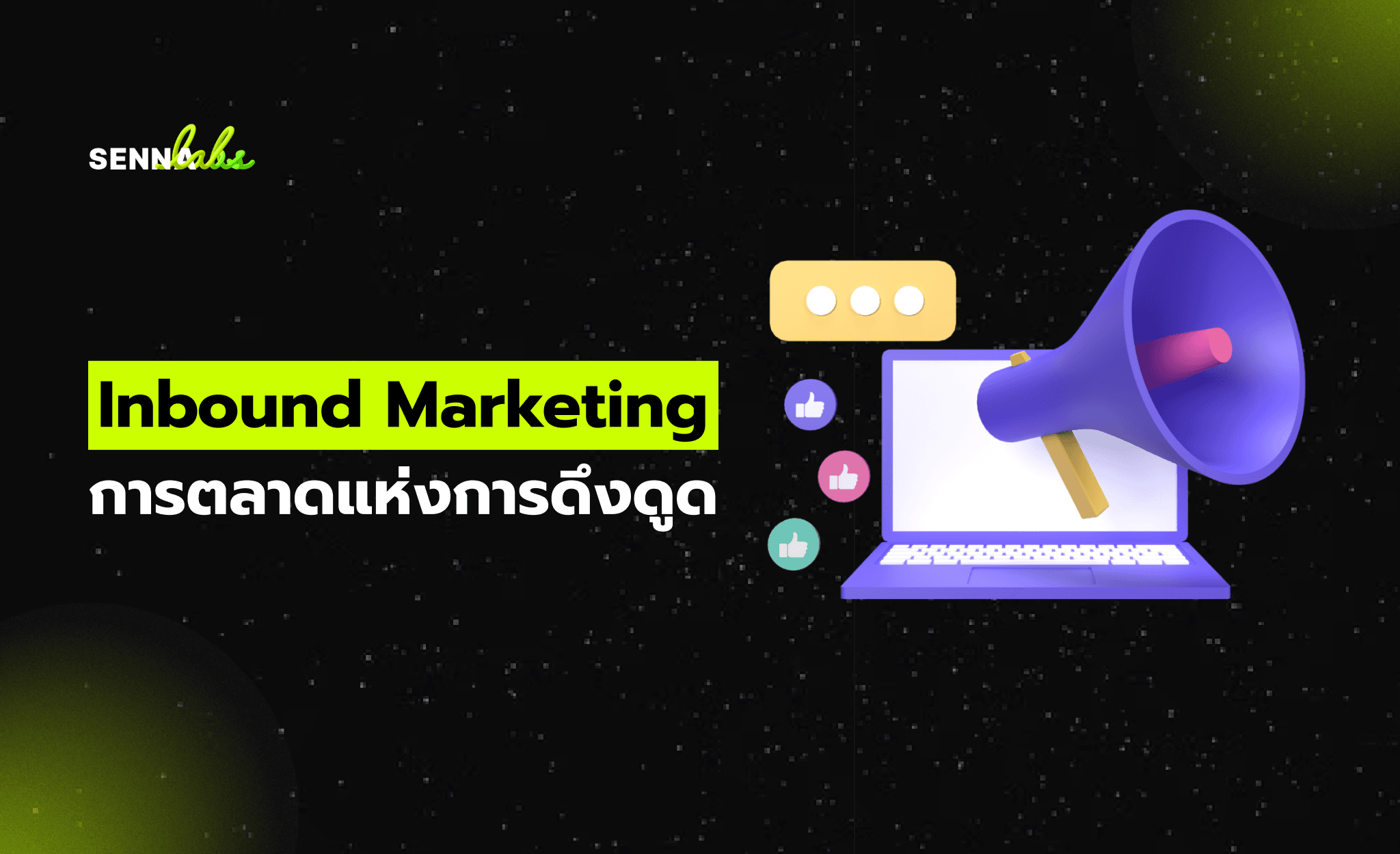
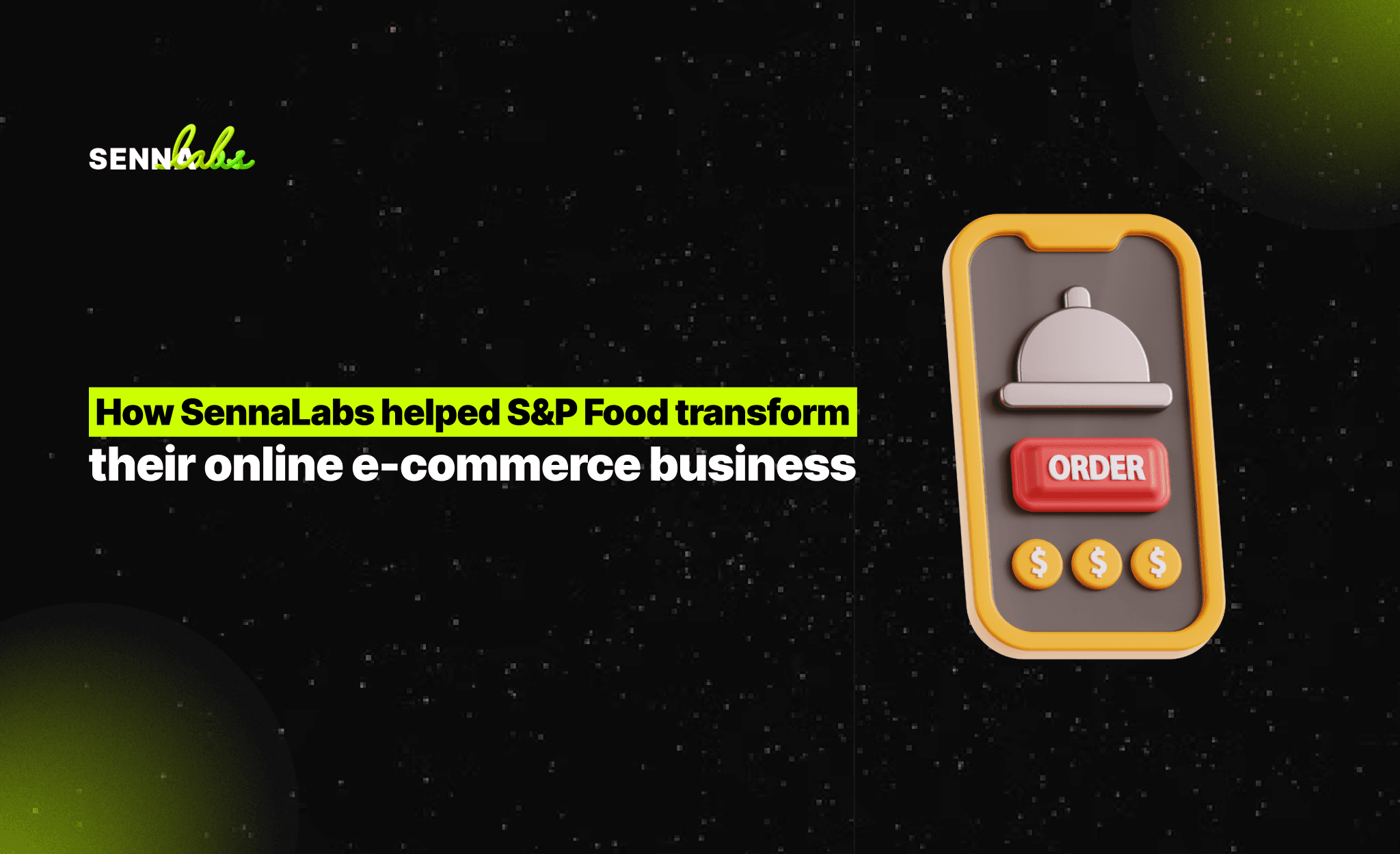
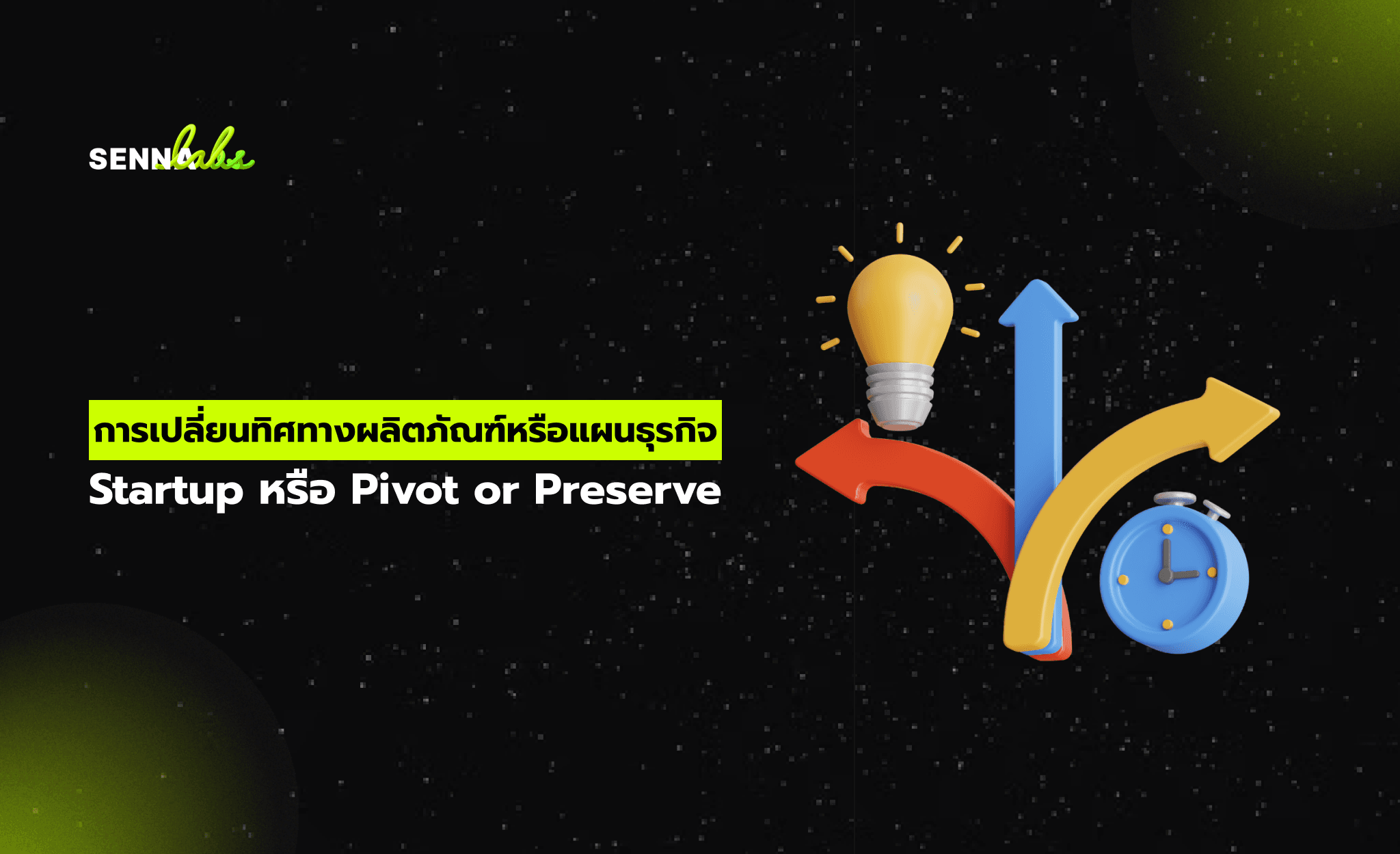
Let’s build digital products that are simply awesome !
We will get back to you within 24 hours!Go to contact us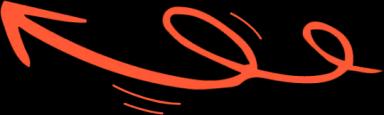
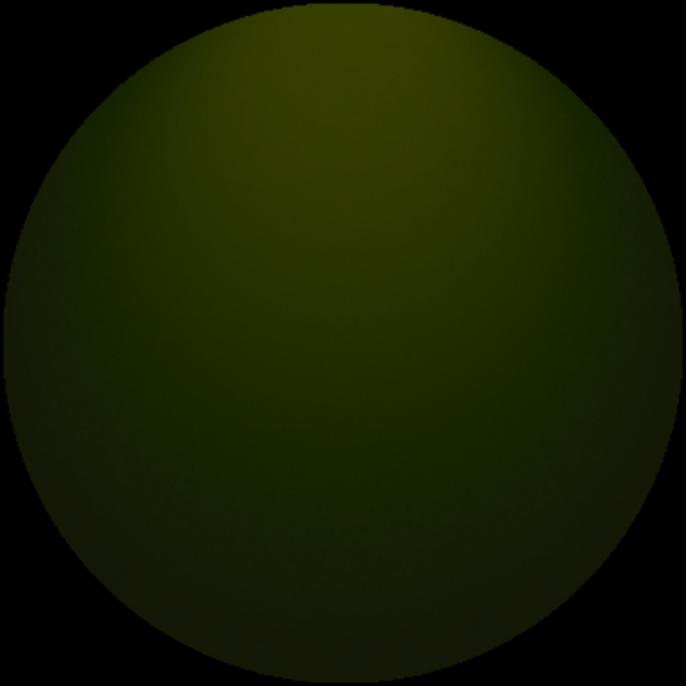
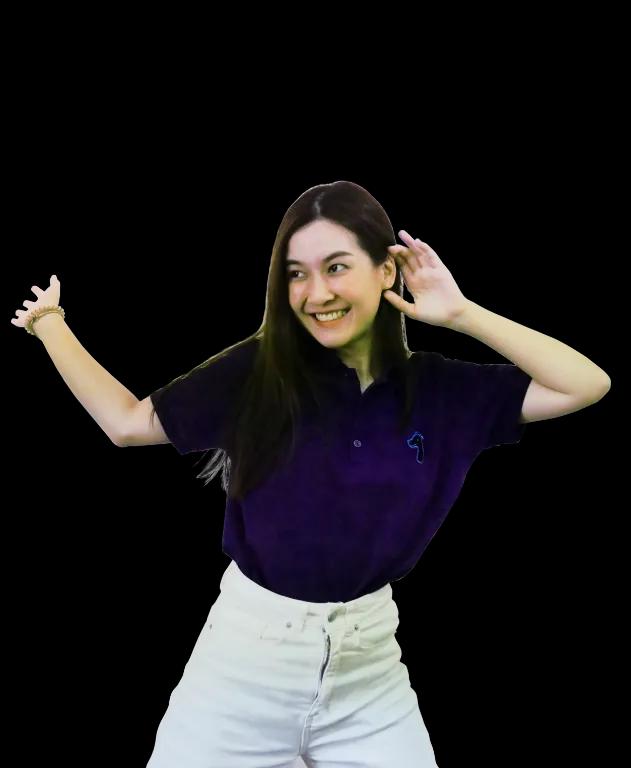
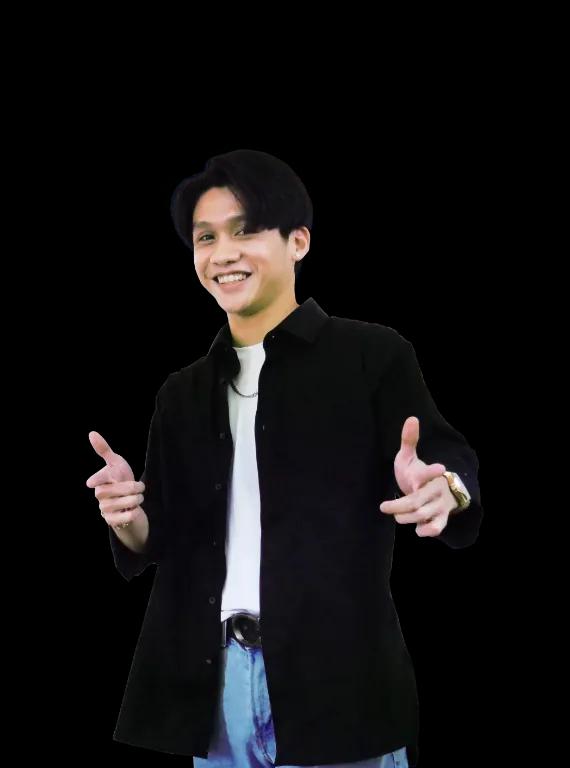
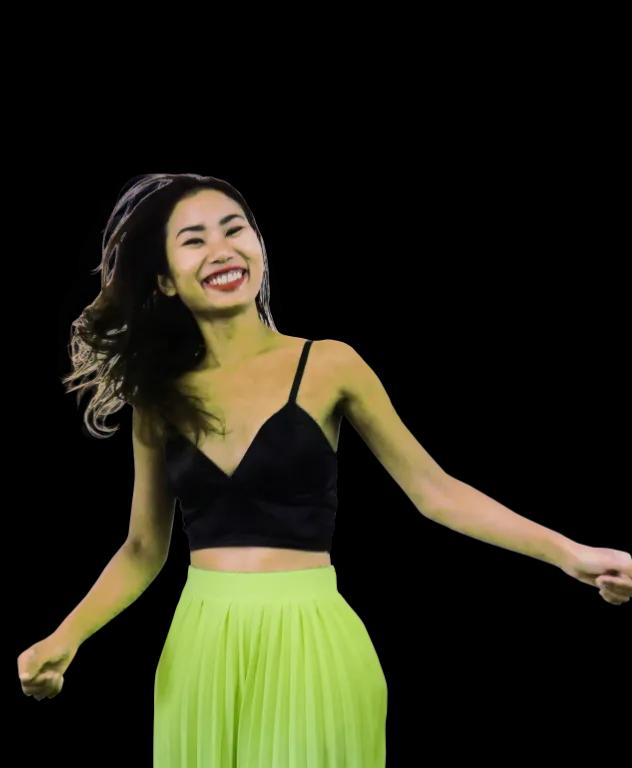
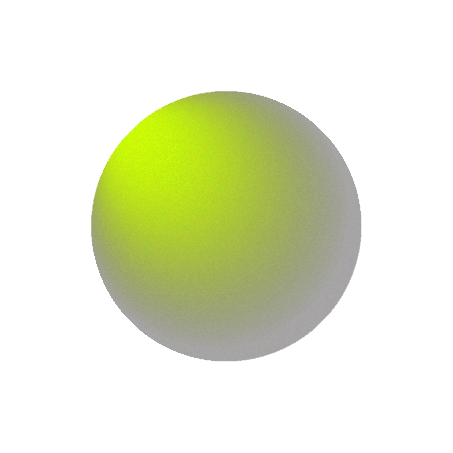
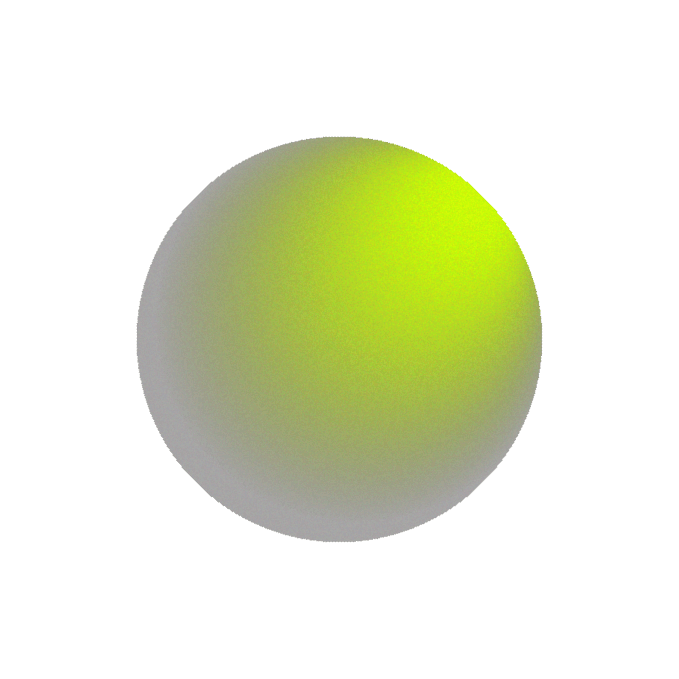
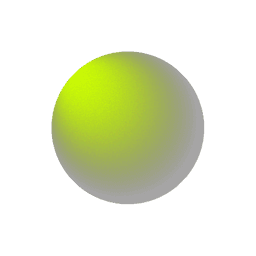
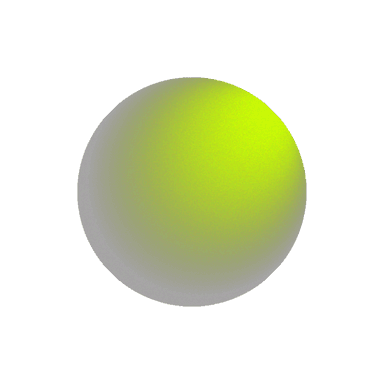
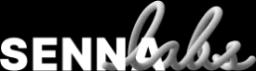