AI Agents and the Right to Explanation: Legal Obligations for Transparency
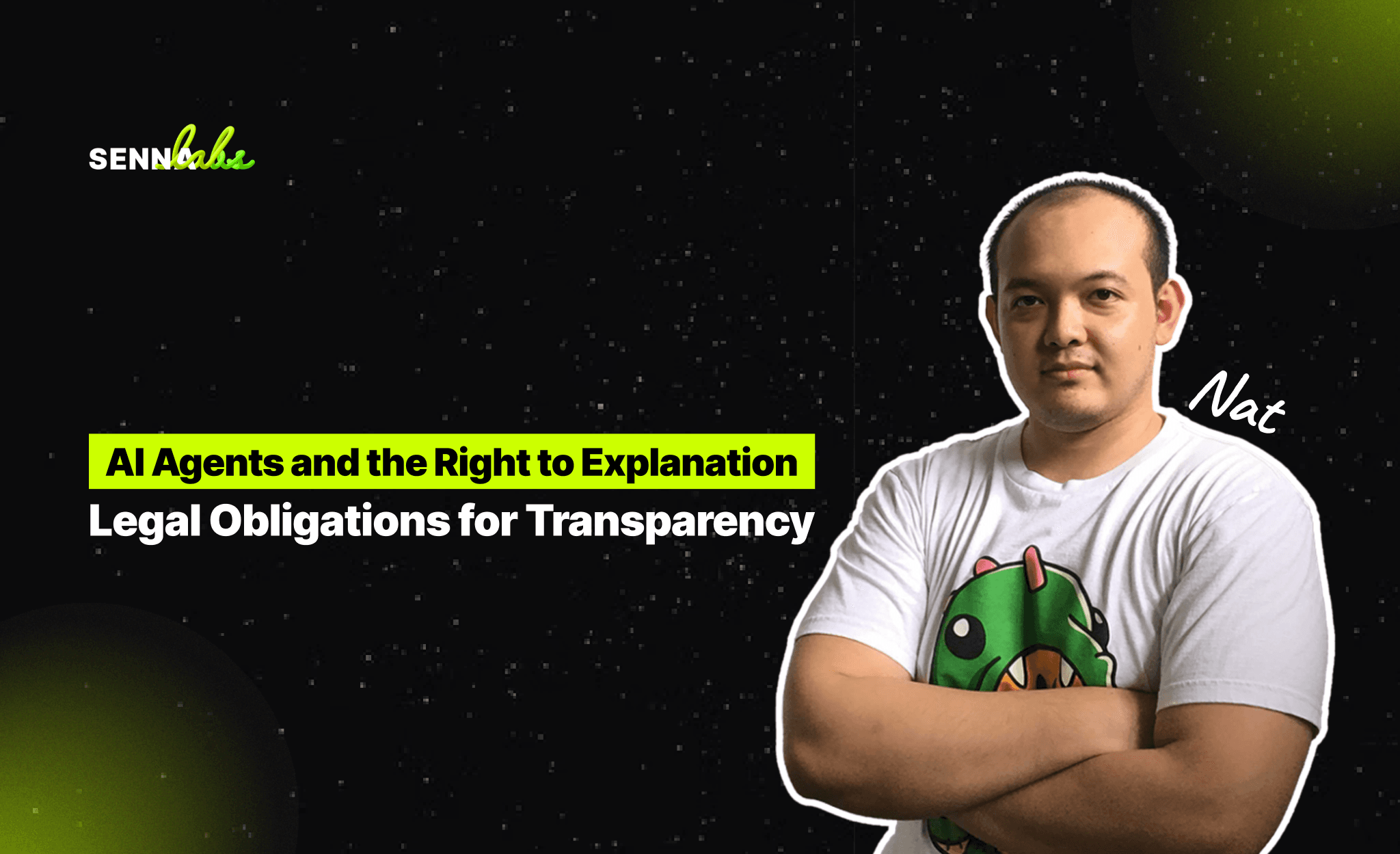
As Artificial Intelligence (AI) systems become increasingly integrated into decision-making processes, transparency has emerged as a critical legal and ethical requirement. The right to an explanation, enshrined in laws such as the General Data Protection Regulation (GDPR), demands that businesses provide clear and understandable reasons for decisions made by AI systems. This obligation ensures accountability, fairness, and trust in AI-driven processes, particularly in high-stakes areas such as credit scoring, hiring, and healthcare.
The Right to Explanation Under GDPR
The GDPR, implemented by the European Union, aims to protect individuals’ data rights and ensure transparency in automated decision-making. Articles 13-15 of the GDPR specifically address the rights of individuals to:
-
Understand Automated Decisions: Individuals must be informed when a decision significantly affecting them is made entirely by automated systems.
-
Receive an Explanation: Businesses must provide meaningful information about the logic behind the decision, including the factors and criteria used.
-
Challenge Decisions: Individuals have the right to contest and seek human intervention in automated decisions.
These provisions place significant responsibility on businesses to make their AI systems interpretable and accountable.
Challenges in Ensuring Transparency
Despite the clear legal mandate, achieving transparency in AI systems can be complex:
-
Algorithmic Complexity: Many AI models, especially those based on deep learning, operate as "black boxes," making their decision-making processes difficult to interpret.
-
Data Dependency: AI decisions often depend on vast datasets that may not be easily explained in a simplified manner.
-
Trade Secrets vs. Transparency: Businesses may hesitate to disclose AI algorithms to protect intellectual property, creating tension with the need for transparency.
-
Lack of Expertise: Not all businesses have the technical expertise to interpret and explain AI decisions effectively to end-users.
Use Case: Fintech Startup Violates GDPR
Situation:
A fintech startup implements an AI credit-scoring system to evaluate loan applications. The system processes applicants’ financial data and automatically approves or declines loans. However, applicants whose loans are declined are not provided with clear explanations for the decision. The reasoning behind scores, such as income thresholds or spending patterns, remains opaque.
Legal Consequences:
Affected individuals file complaints, and regulators find the startup in violation of GDPR’s transparency requirements. The company is fined for failing to provide meaningful explanations and is required to overhaul its AI system to ensure compliance.
Lessons Learned:
-
Businesses must design AI systems with explainability in mind.
-
Transparency mechanisms should be embedded from the start to avoid legal risks.
-
Users must be provided with clear, non-technical explanations for decisions affecting them.
Ensuring AI Transparency: Best Practices
1. Implement Explainable AI (XAI)
Businesses should prioritize Explainable AI frameworks that simplify the decision-making process into understandable insights. Tools such as SHAP (Shapley Additive Explanations) or LIME (Local Interpretable Model-Agnostic Explanations) can make AI outputs interpretable.
2. Provide Layered Explanations
Different users may require varying levels of explanation. For example:
-
End-users: Simplified explanations of why a decision was made.
-
Regulators: Detailed technical descriptions of AI logic and data sources.
3. Maintain Decision Logs
AI systems should record the data, criteria, and logic used in each decision to provide a clear audit trail. This ensures accountability and supports compliance in case of disputes.
4. Design for Human Oversight
Businesses should incorporate mechanisms for human intervention in automated decisions, especially in sensitive areas such as credit scoring or hiring.
5. Train Staff on AI Ethics
Employees should be trained to understand the ethical and legal responsibilities of using AI, including the right to explanation and transparency obligations.
Benefits of Transparency in AI
-
Compliance with Regulations: Meeting legal requirements avoids penalties and enhances credibility with regulators.
-
Building Consumer Trust: Transparent AI systems foster confidence among users, leading to higher engagement and satisfaction.
-
Improved Decision Quality: Explainability helps businesses identify and address biases or errors in AI systems, improving their overall reliability.
-
Competitive Advantage: Transparency can differentiate businesses in the market, positioning them as responsible and customer-focused.
Future of AI Transparency
As regulations like GDPR evolve and new frameworks are introduced worldwide, businesses must stay ahead of the curve by adopting proactive transparency practices. Emerging technologies, such as Explainable AI (XAI) and Responsible AI frameworks, will play a pivotal role in enabling businesses to meet their legal and ethical obligations.
Conclusion
The right to explanation is more than just a regulatory requirement—it’s a cornerstone of responsible AI use. Businesses that fail to provide transparency risk not only legal penalties but also the erosion of consumer trust. By prioritizing explainable AI, clear communication, and compliance with frameworks like GDPR, companies can build ethical, reliable AI systems that benefit both users and organizations.
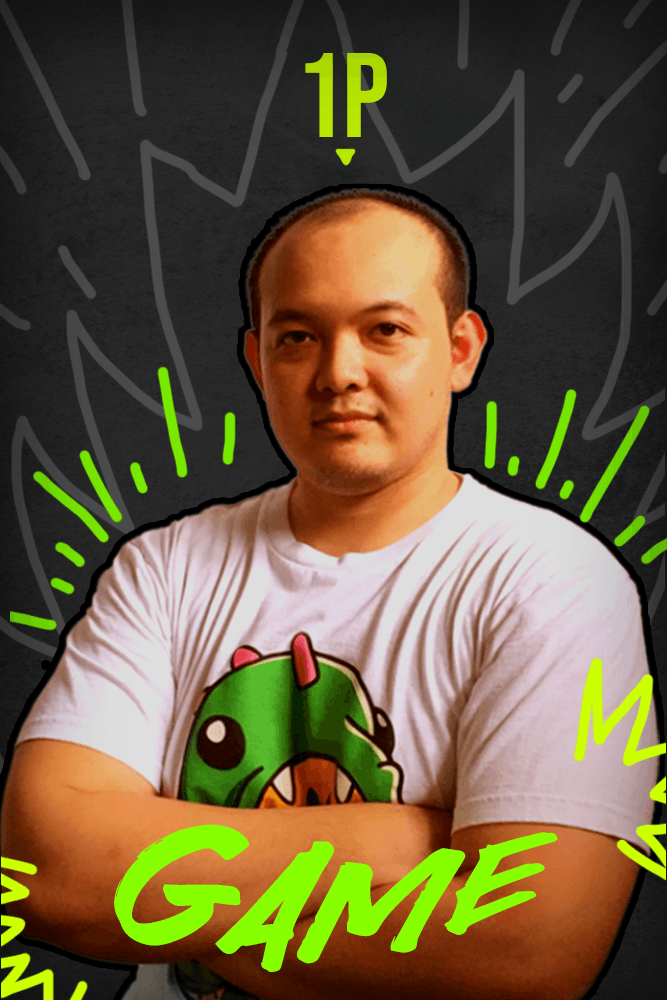
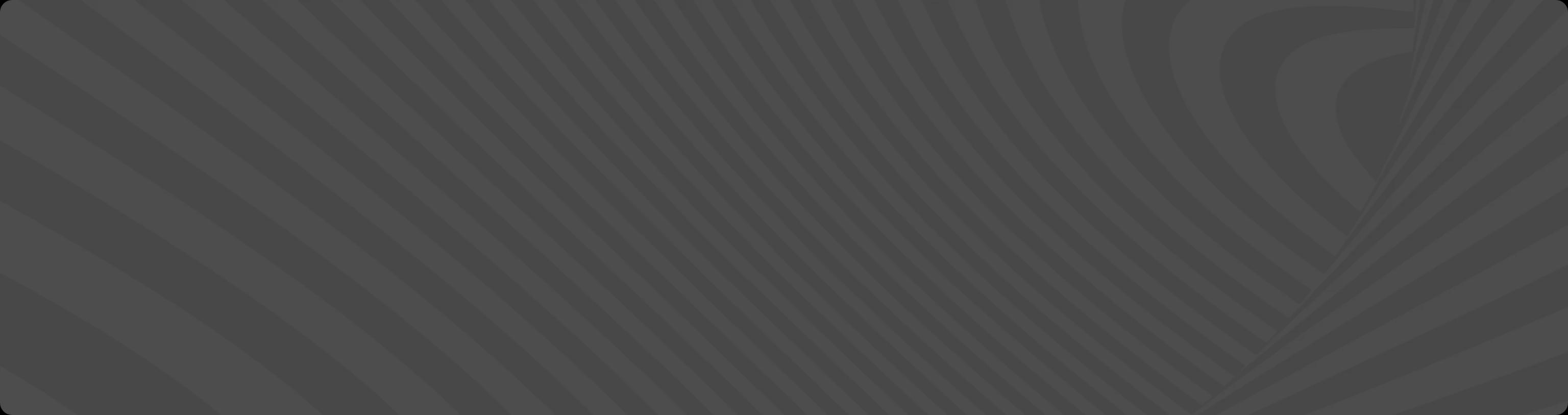
Subscribe to follow product news, latest in technology, solutions, and updates
Other articles for you
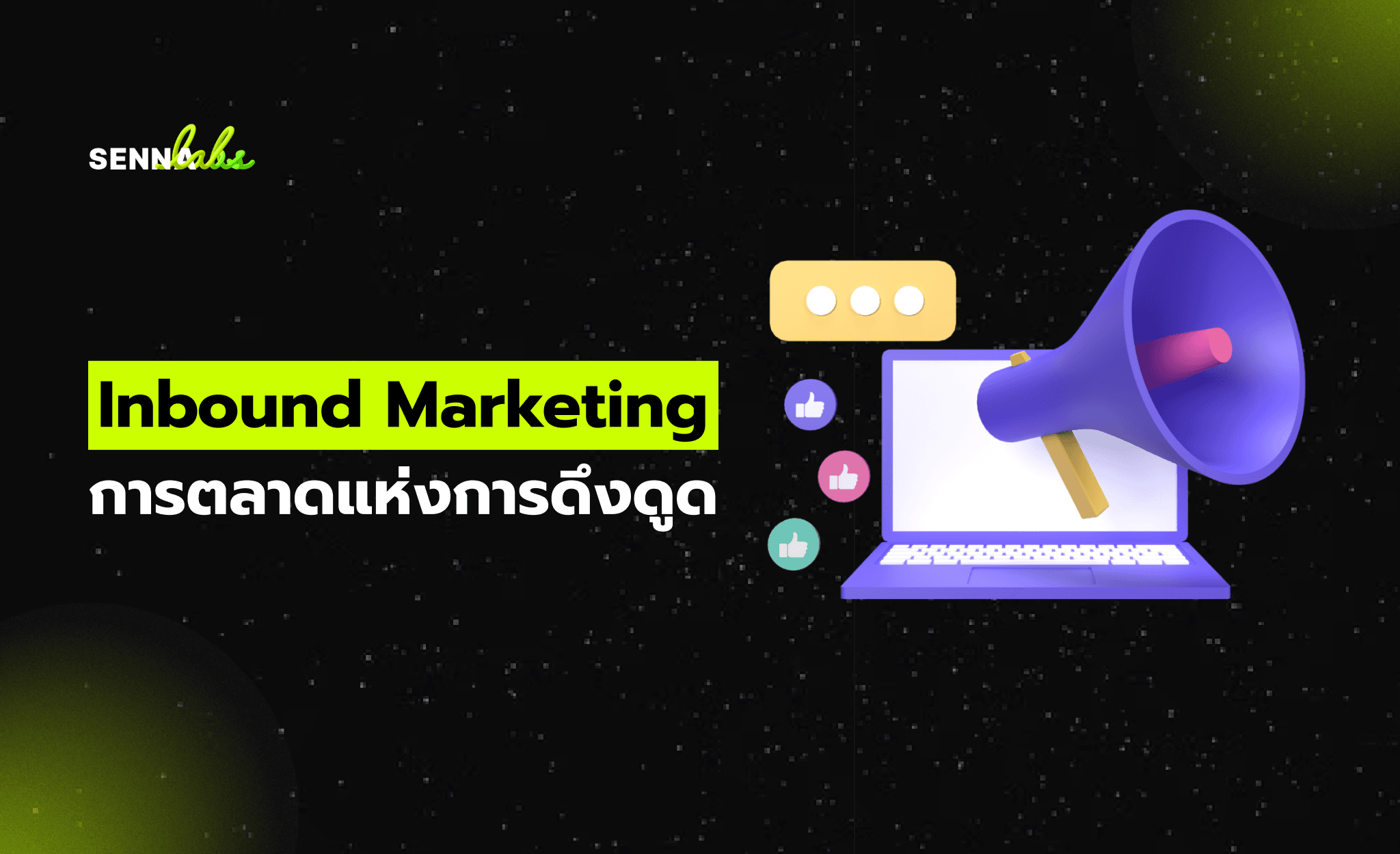
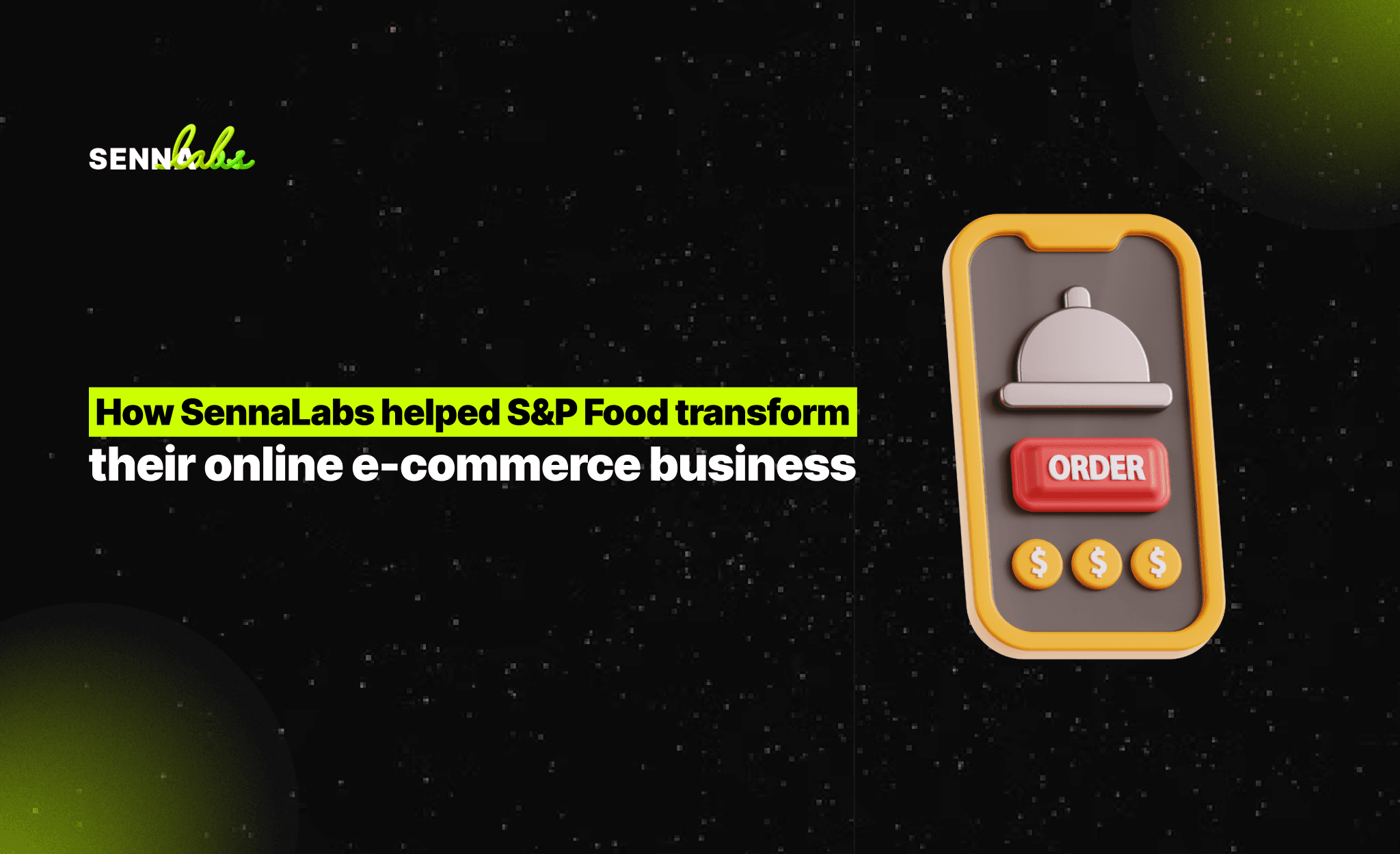
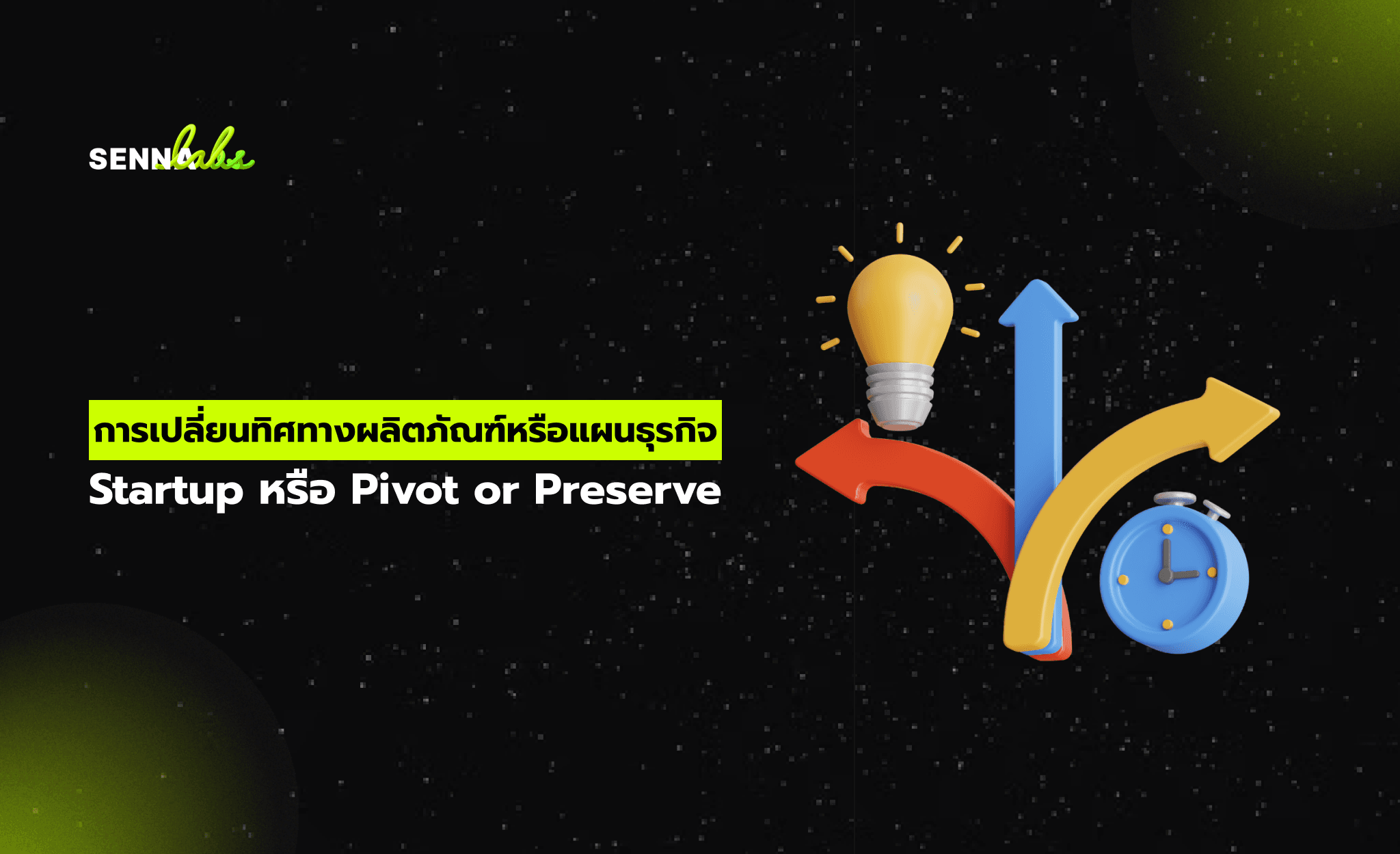
Let’s build digital products that are simply awesome !
We will get back to you within 24 hours!Go to contact us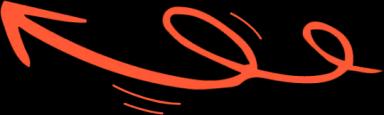
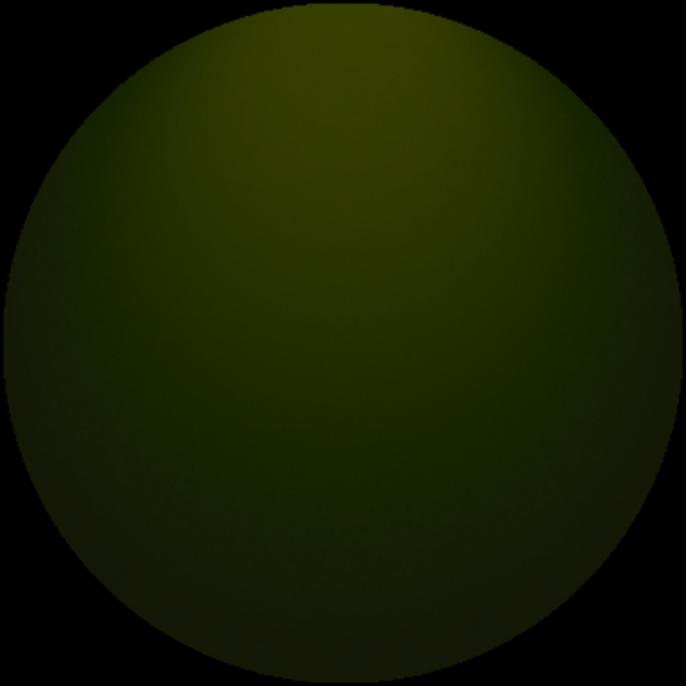
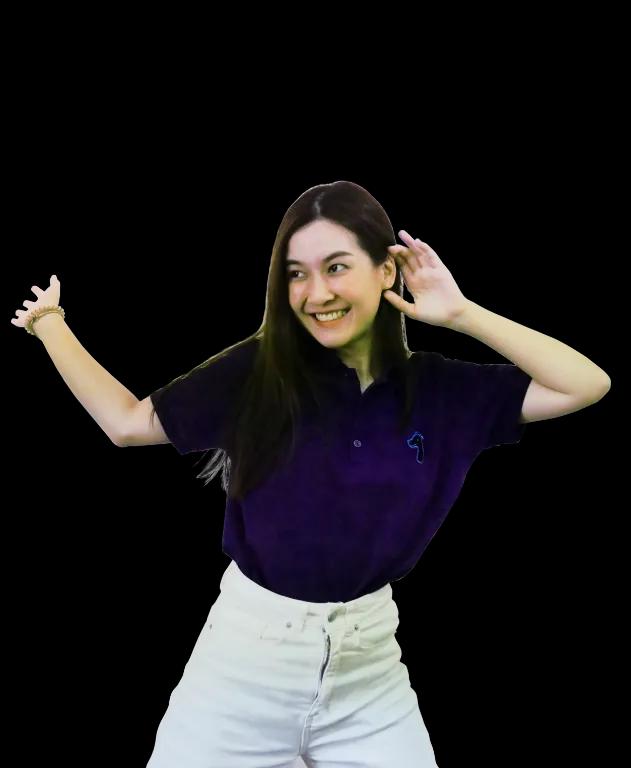
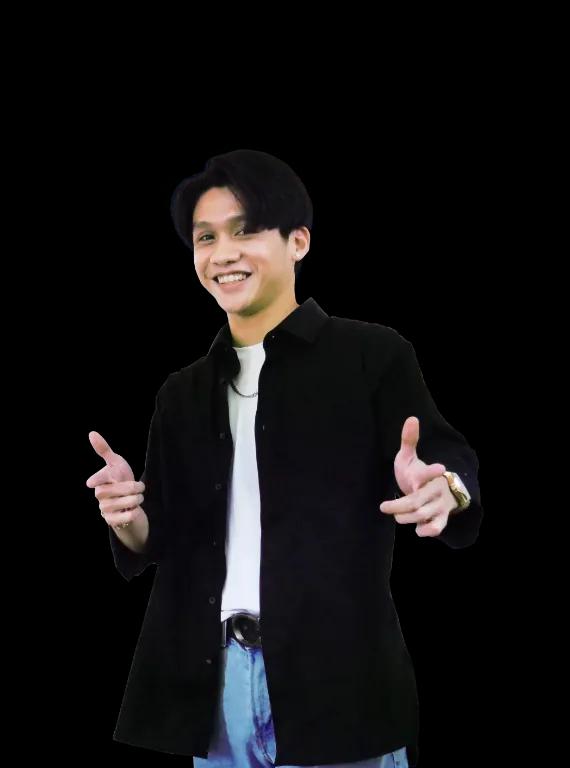
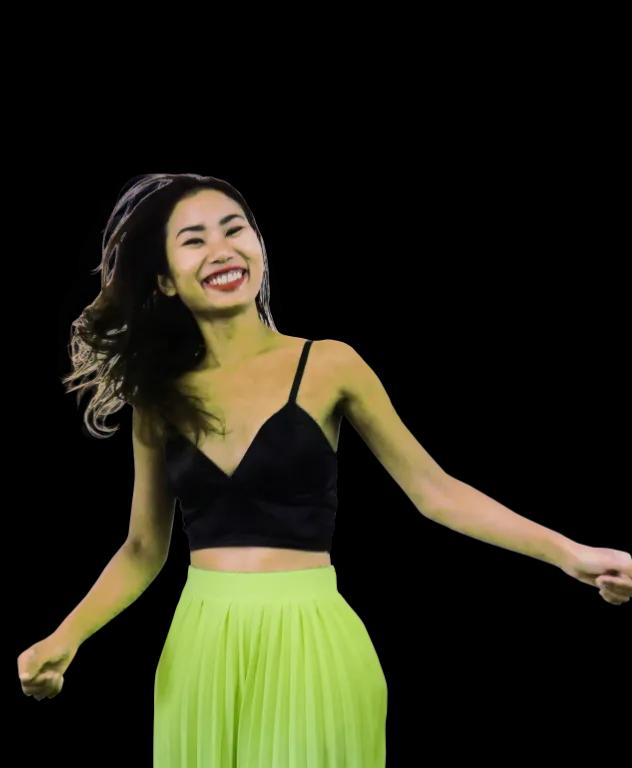
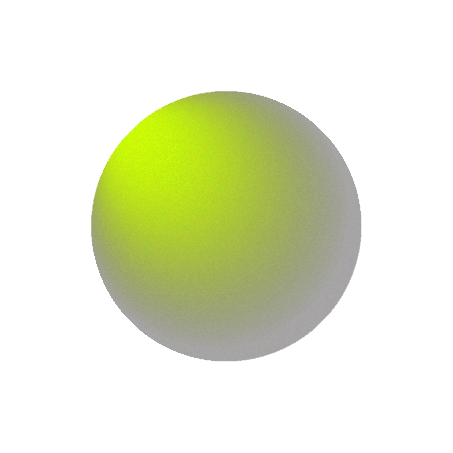
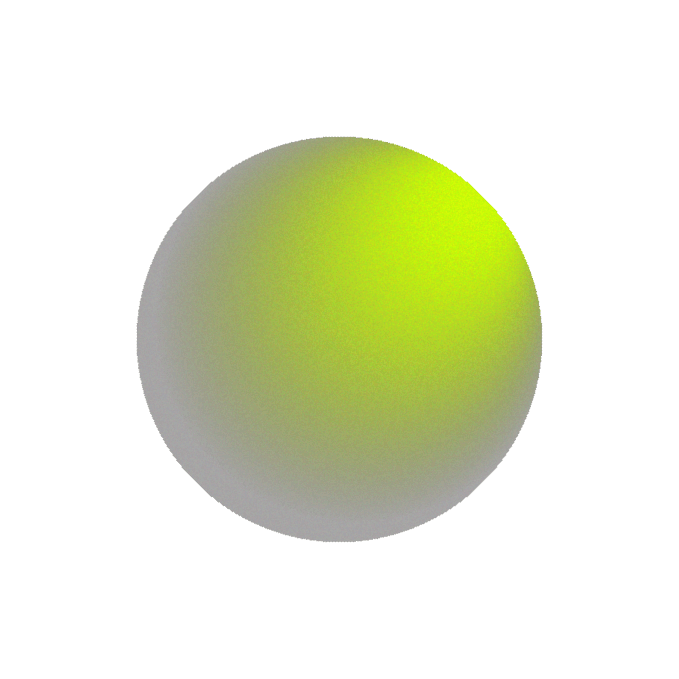
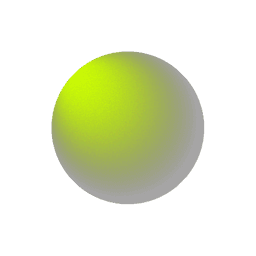
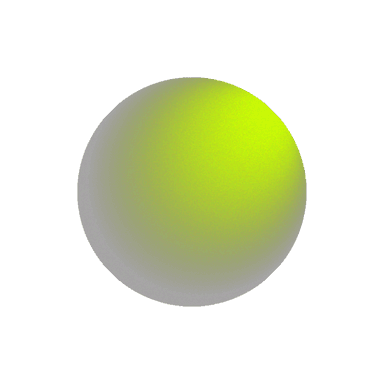
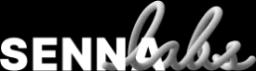