AI in Manufacturing: Selecting Tools for Automation and Efficiency
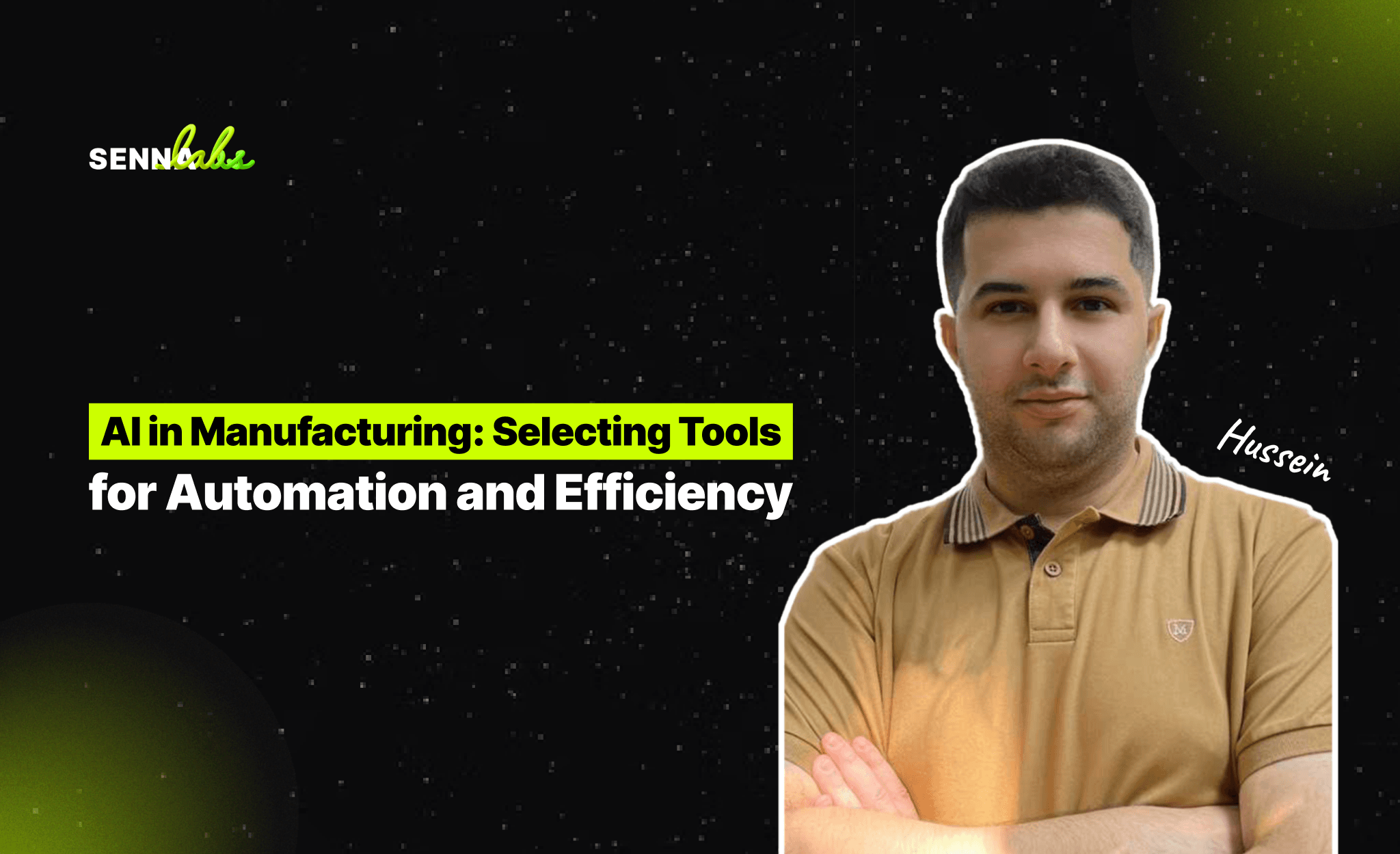
In the fast-paced world of manufacturing, maintaining high efficiency and reducing errors are critical for staying competitive. Artificial Intelligence (AI) is revolutionizing the industry by automating processes, enhancing quality control, and optimizing operations.
This article explores how manufacturers can choose the right AI tools to automate production lines and improve efficiency, focusing on a use case where a factory adopts an AI-powered quality control system to detect defects in real-time.
The Need for AI in Manufacturing
Traditional manufacturing processes face several challenges:
-
Human Error: Manual inspections and processes can lead to inconsistencies and missed defects.
-
Time-Consuming Tasks: Quality control and routine checks consume significant time and resources.
-
Limited Scalability: Scaling operations without sacrificing quality is a common hurdle.
-
Data Utilization: Many factories collect data but lack the tools to analyze and act on it effectively.
AI tools address these challenges by automating repetitive tasks, providing real-time insights, and enabling manufacturers to operate more efficiently.
Benefits of AI in Manufacturing
Adopting AI in manufacturing offers numerous advantages:
-
Improved Quality Control: AI systems detect defects with greater accuracy than manual inspections.
-
Increased Efficiency: Automated workflows reduce downtime and optimize resource allocation.
-
Cost Savings: Reducing errors and waste leads to significant cost reductions.
-
Predictive Maintenance: AI predicts equipment failures, minimizing unplanned downtime.
-
Scalability: AI tools adapt to growing production demands without compromising quality.
Steps to Select the Right AI Tools for Manufacturing
Step 1: Identify Business Goals
Clearly define what you want to achieve with AI. Common goals include:
-
Defect Detection: Identifying flaws in products during production.
-
Process Optimization: Improving throughput and reducing cycle times.
-
Predictive Maintenance: Anticipating equipment failures before they occur.
Example: A factory aims to reduce product defects and improve overall quality control.
Step 2: Evaluate Data Readiness
AI relies on data for training and decision-making. Assess your data:
-
Data Availability: Do you have enough historical and real-time data from sensors, cameras, or production logs?
-
Data Quality: Is your data clean, structured, and accurate?
-
Data Storage: Do you have the infrastructure to store and manage large datasets?
Example: The factory uses production line cameras to collect image data for defect analysis.
Step 3: Research AI Tools and Providers
Explore AI tools and vendors that align with your goals. Key considerations include:
-
Functionality: Does the tool address your specific needs, such as defect detection or predictive maintenance?
-
Integration: Can the AI system integrate seamlessly with your existing equipment and software?
-
Ease of Use: Is the system user-friendly, or does it require extensive training?
-
Scalability: Can the tool adapt to future production increases or additional use cases?
Example: The factory evaluates AI-powered quality control platforms like Landing AI and Vanti Analytics for real-time defect detection.
Step 4: Pilot the AI System
Before full-scale deployment, test the AI tool in a controlled environment:
-
Set Up a Pilot Project: Use the AI system on one production line or a specific product.
-
Measure Results: Track metrics like defect detection rate, cycle time, and cost savings.
-
Collect Feedback: Involve operators and technicians to identify any usability issues.
Example: The factory pilots the quality control AI on a production line for electronic components, achieving a 30% improvement in defect detection accuracy.
Step 5: Deploy and Monitor the System
Once the AI tool proves effective in the pilot phase, deploy it across the factory:
-
Integrate System-Wide: Connect the AI system to all production lines and relevant software platforms.
-
Monitor Performance: Use dashboards and reports to track key performance indicators (KPIs).
-
Continuous Improvement: Update the AI model with new data to maintain accuracy and efficiency.
Example: The factory implements the AI tool factory-wide, reducing defect rates by 25% and saving $200,000 annually.
Use Case: AI-Powered Quality Control
Problem:
A factory producing consumer electronics struggled with inconsistent quality due to manual inspections, leading to increased returns and customer dissatisfaction.
Solution:
The factory implemented an AI-powered quality control system that used computer vision to analyze each product on the production line.
Results:
-
Defect Detection: The AI system identified defects in real-time, such as cracks and misalignments.
-
Improved Efficiency: Automated inspections reduced the time spent on manual checks by 40%.
-
Cost Savings: Waste and rework decreased significantly, saving the factory $500,000 in the first year.
Examples of AI Tools for Manufacturing
-
Landing AI: Specialized in computer vision for quality control.
-
IBM Watson IoT: Offers predictive maintenance and process optimization.
-
Bright Machines: Focuses on automating manufacturing workflows.
-
Vanti Analytics: Provides actionable insights to optimize production and detect anomalies.
-
TensorFlow and PyTorch: Open-source libraries for building custom AI models.
Challenges and How to Overcome Them
Challenge 1: High Initial Costs
Solution: Start with pilot projects to measure ROI before scaling up.
Challenge 2: Data Privacy Concerns
Solution: Use secure systems that comply with data protection regulations.
Challenge 3: Resistance to Change
Solution: Provide training and demonstrate the benefits of AI to employees.
The Future of AI in Manufacturing
The integration of AI in manufacturing is poised to grow, with trends including:
-
Autonomous Factories: Fully automated operations with minimal human intervention.
-
Sustainability: AI optimizing energy use and reducing waste.
-
Edge AI: Real-time processing at the production site for faster decision-making.
-
AI-Driven Design: Using AI to create and test product designs before production.
Conclusion
AI is transforming manufacturing by automating processes, improving quality, and boosting efficiency. By following a structured approach to selecting AI tools, manufacturers can address specific challenges and unlock significant benefits.
For the factory adopting an AI-powered quality control system, the results are clear: reduced defects, cost savings, and improved customer satisfaction. As AI technology continues to evolve, its potential to revolutionize manufacturing grows, offering endless opportunities for innovation and growth.

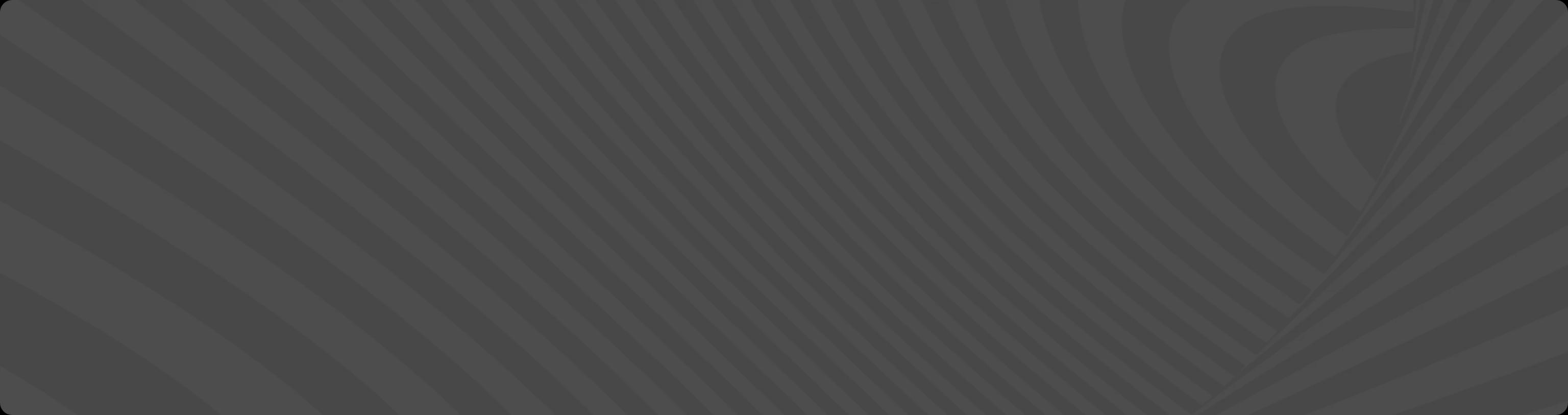
Subscribe to follow product news, latest in technology, solutions, and updates
Other articles for you
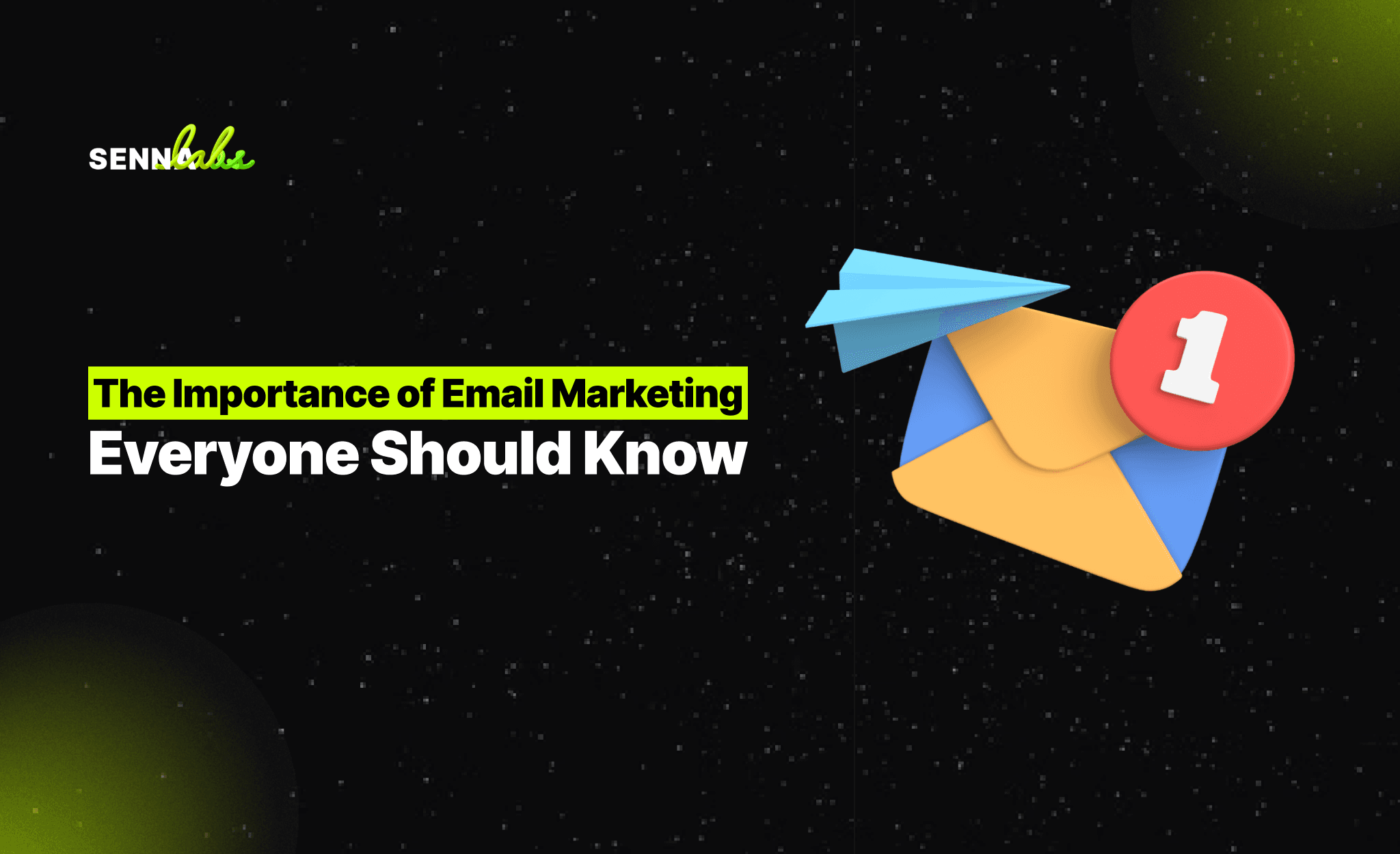
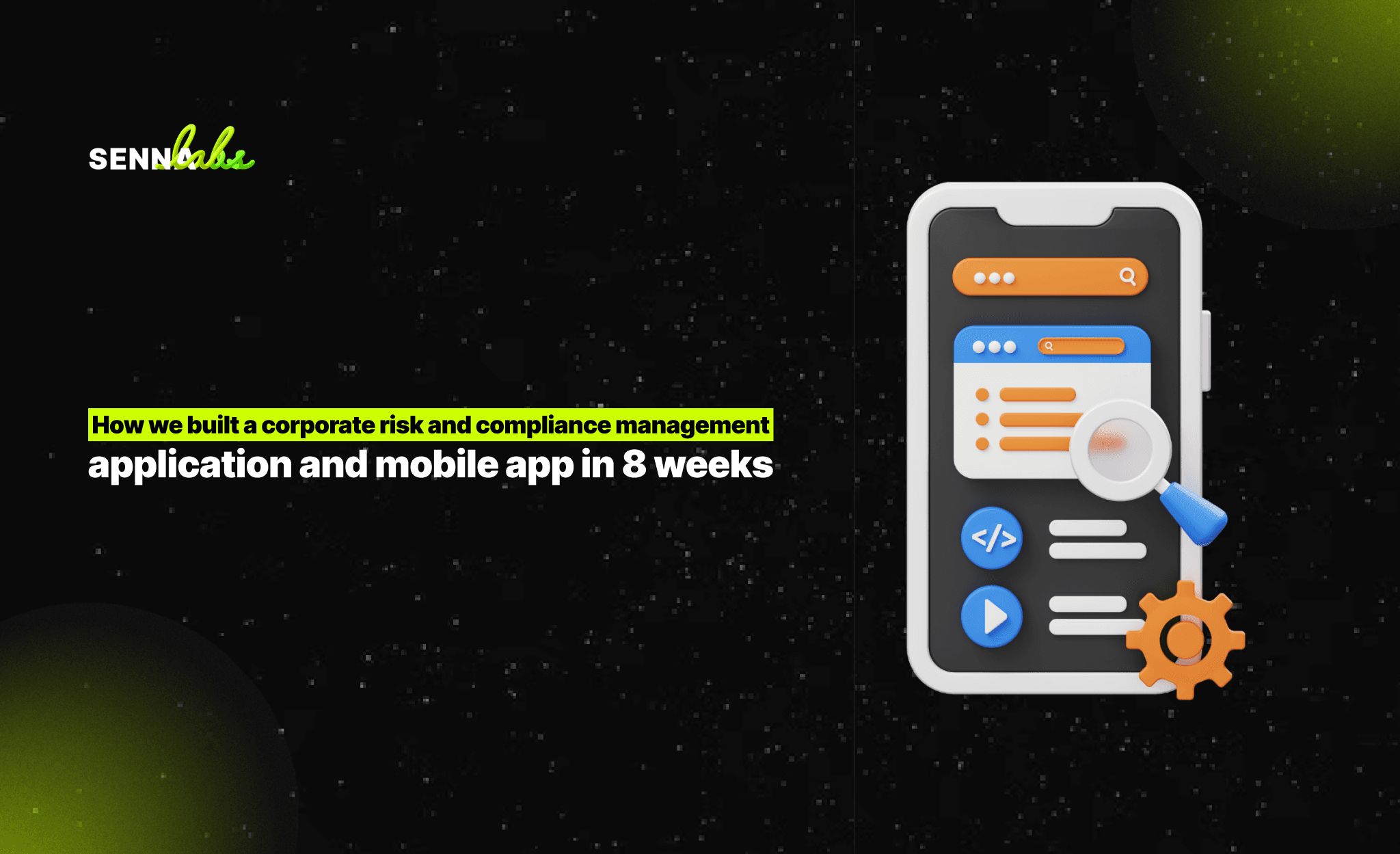
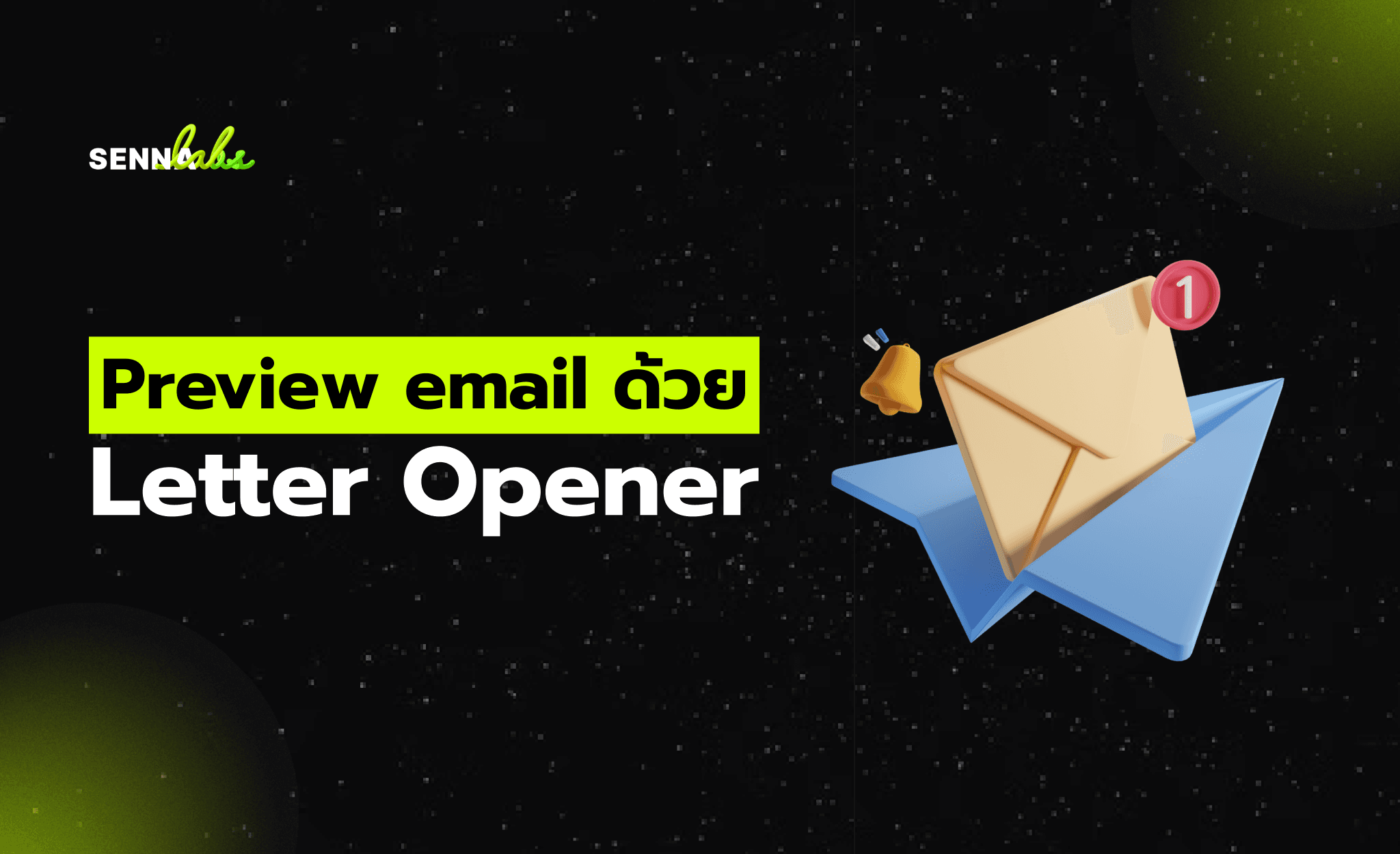
Let’s build digital products that are simply awesome !
We will get back to you within 24 hours!Go to contact us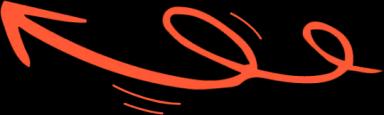
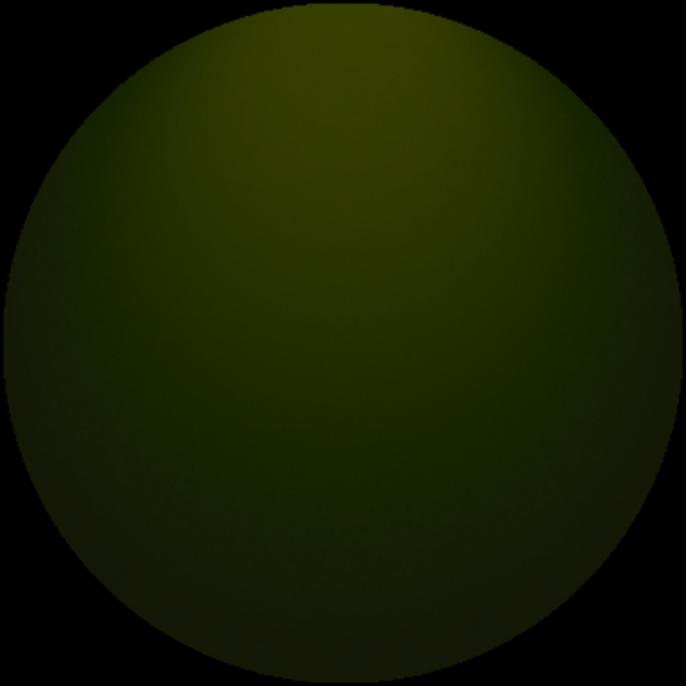
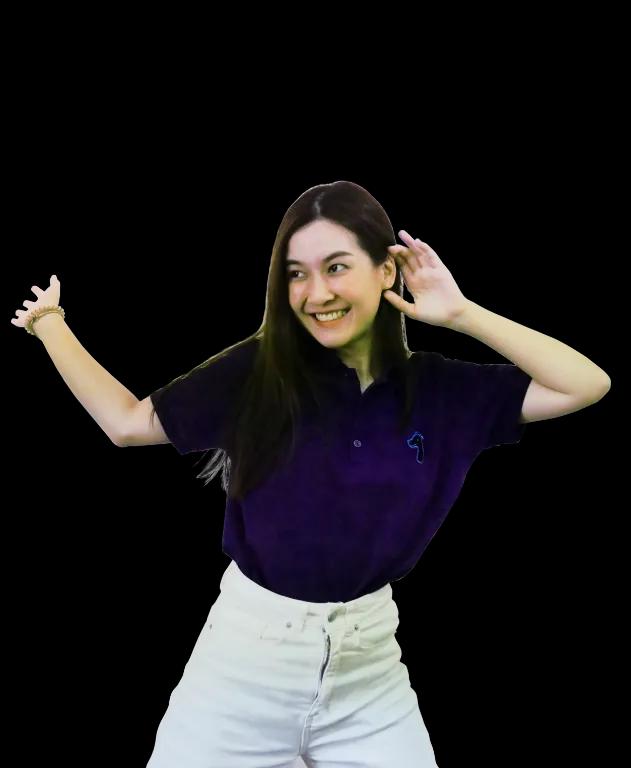
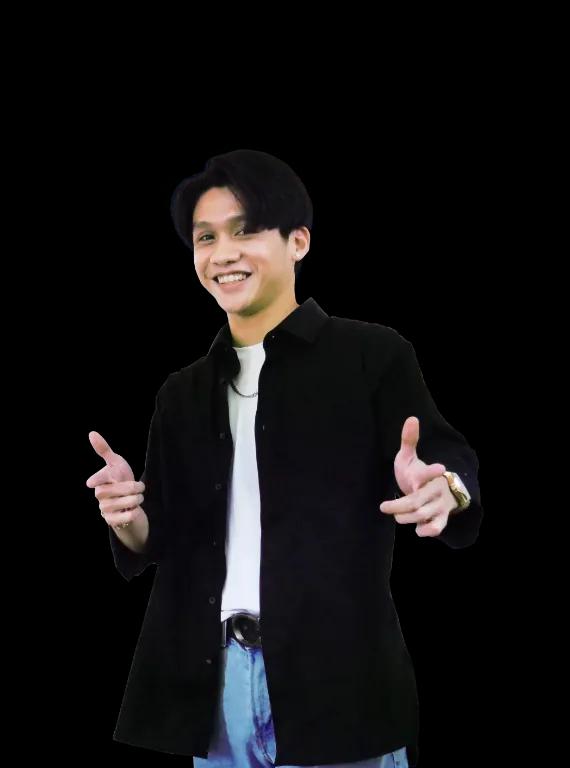
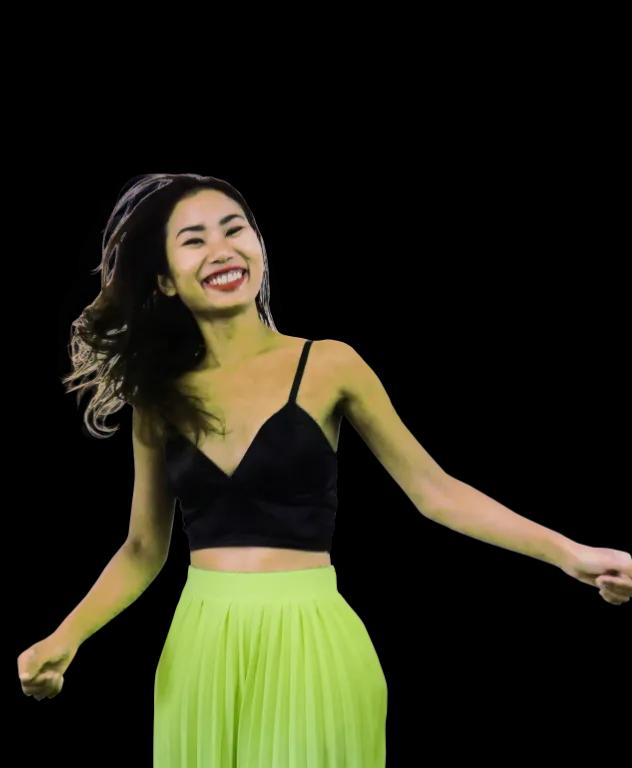
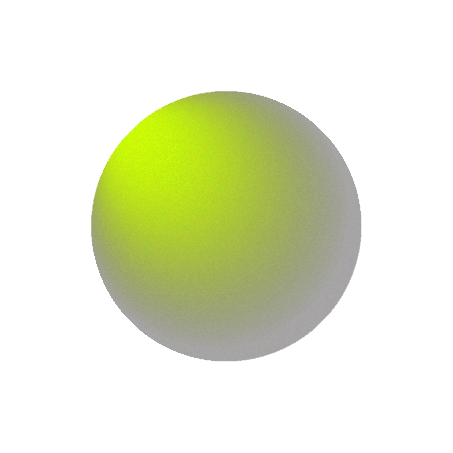
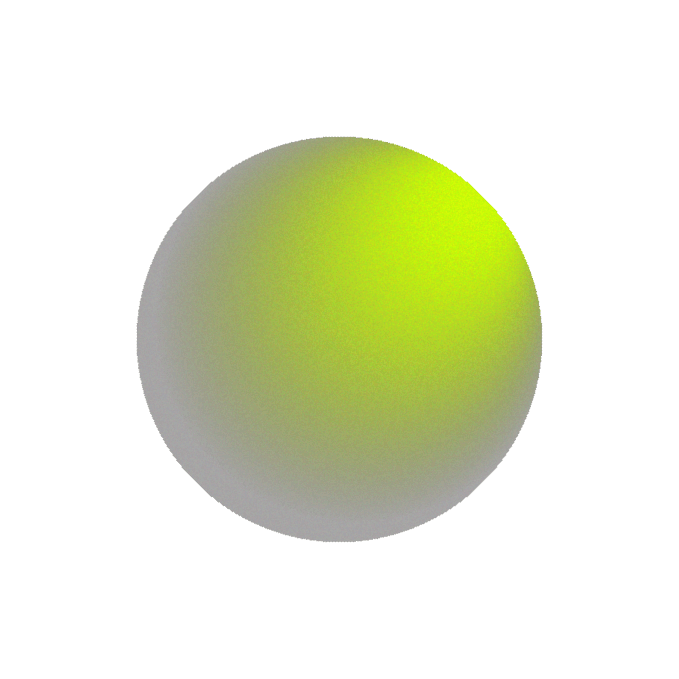
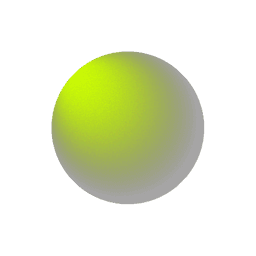
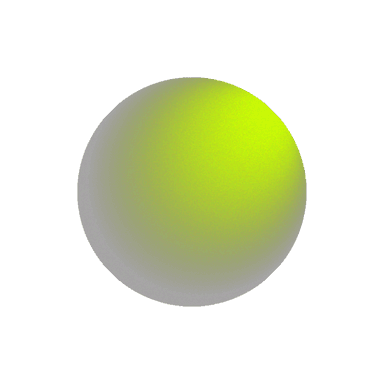
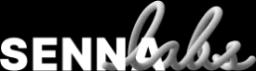