How Machine Learning Enhances Logistics Operations with AI Agents
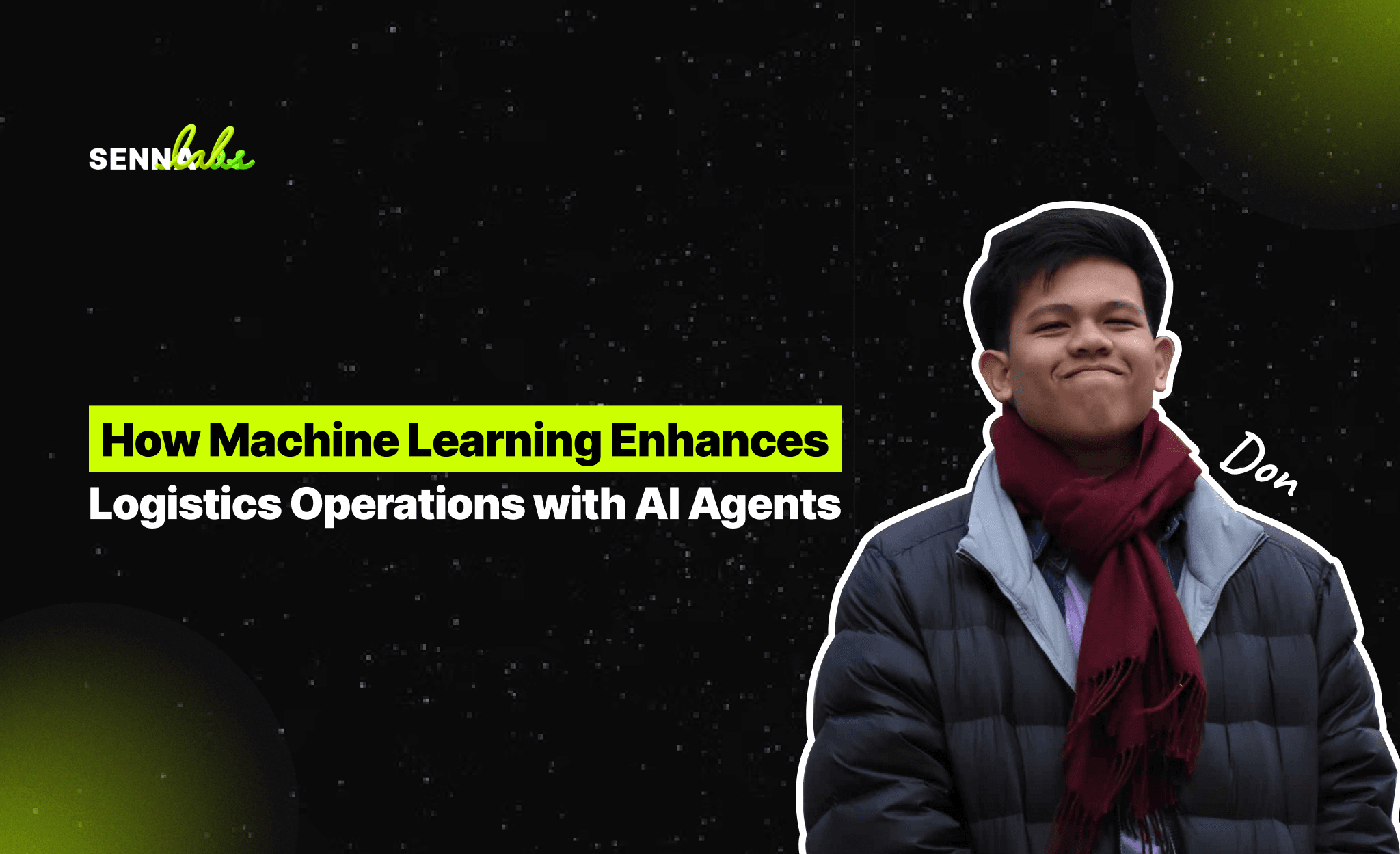
Logistics is the backbone of modern commerce, ensuring that goods reach their destinations efficiently. However, challenges such as unpredictable traffic, fluctuating demand, and the need for timely deliveries make logistics management a complex task. AI agents powered by machine learning are stepping in to revolutionize logistics by predicting delivery times and optimizing routes, improving efficiency and reducing costs.
This article explores how machine learning algorithms enable AI agents to analyze real-time traffic data, predict delivery timelines, and minimize delays, transforming logistics operations.
The Challenge: Navigating Complex Logistics
Logistics companies face several challenges in ensuring smooth operations:
-
Unpredictable Traffic: Sudden traffic jams, road closures, or accidents can disrupt delivery schedules.
-
Inefficient Routing: Without real-time optimization, routes may be unnecessarily long, increasing fuel consumption and delivery times.
-
Customer Expectations: The rise of same-day and next-day delivery demands has made timeliness critical.
-
Resource Allocation: Managing vehicles and drivers efficiently is a constant struggle.
Traditional methods struggle to address these issues in dynamic, fast-changing environments.
The Solution: AI Agents in Logistics
AI agents, powered by machine learning, bring intelligence and adaptability to logistics operations. Here’s how they transform the process:
-
Real-Time Data Analysis: AI agents analyze traffic data, weather conditions, and delivery locations in real time to identify potential disruptions.
-
Route Optimization: Using machine learning algorithms, AI agents calculate the most efficient routes, considering factors like traffic density, road conditions, and fuel efficiency.
-
Delivery Time Prediction: AI agents predict accurate delivery times by analyzing historical data and current conditions, keeping customers informed and satisfied.
-
Dynamic Adjustments: If unexpected events occur, such as a roadblock, AI agents reroute deliveries instantly to avoid delays.
-
Resource Allocation: AI agents help allocate vehicles and drivers efficiently, ensuring optimal utilization of resources.
Use Case: AI Agents in a Logistics Company
Imagine a logistics company using AI agents to optimize its delivery operations. Here’s how it works:
Step 1: Analyzing Real-Time Data
-
An AI agent monitors traffic data from IoT sensors, GPS devices, and public traffic feeds.
-
It identifies a traffic jam on a major highway that affects several scheduled deliveries.
Step 2: Optimizing Routes
-
The AI agent recalculates the routes for affected drivers, redirecting them to less congested roads.
-
It also considers fuel efficiency, ensuring the new routes minimize additional costs.
Step 3: Predicting Delivery Times
-
The AI agent updates the delivery timelines for all affected orders and informs customers of the adjusted times.
Step 4: Dynamic Resource Allocation
-
If a driver is delayed, the AI agent reallocates another vehicle nearby to ensure critical deliveries are not missed.
Benefits of AI Agents in Logistics
Integrating AI agents into logistics operations provides numerous advantages:
-
Improved Efficiency: Real-time route optimization reduces fuel consumption, delivery times, and costs.
-
Enhanced Customer Satisfaction: Accurate delivery predictions and proactive updates build trust and loyalty.
-
Reduced Delays: AI agents quickly adapt to disruptions, minimizing the impact on delivery schedules.
-
Better Resource Utilization: Vehicles and drivers are allocated effectively, maximizing productivity.
-
Scalability: AI systems can manage logistics operations for companies of all sizes, from local couriers to global shipping networks.
The Role of Machine Learning
Machine learning algorithms are the foundation of effective AI agents in logistics. These algorithms:
-
Analyze Patterns: Study historical delivery data to identify trends and predict future delays.
-
Optimize Continuously: Learn from past performance to improve routing and prediction accuracy over time.
-
Incorporate Multiple Data Sources: Combine traffic, weather, and operational data to make informed decisions.
This adaptive capability ensures that AI agents remain relevant and effective in dynamic logistics environments.
Challenges and Solutions
While AI agents offer transformative potential, their implementation comes with challenges:
-
Data Quality: Inaccurate or incomplete data can hinder AI performance.
Solution: Use reliable data sources and continuously validate and update datasets. -
Integration Costs: Implementing AI systems requires upfront investment.
Solution: Start with scalable AI solutions that can grow with the business. -
Resistance to Change: Drivers and staff may hesitate to adopt new technology.
Solution: Provide training and demonstrate the benefits of AI-driven operations.
The Future of Logistics with AI
As AI agents and machine learning continue to evolve, the future of logistics looks smarter and more efficient. Emerging trends include:
-
Autonomous Deliveries: AI agents guiding autonomous vehicles and drones for faster, more reliable deliveries.
-
Predictive Logistics: Anticipating demand patterns and preparing resources in advance.
-
Sustainability: Optimizing routes to reduce carbon footprints and fuel consumption.
Conclusion
AI agents powered by machine learning are transforming logistics by optimizing routes, predicting delivery times, and reducing delays. These intelligent systems bring adaptability and precision to an industry where efficiency is critical.
For logistics companies, adopting AI-driven solutions is no longer optional—it’s essential to remain competitive in a rapidly evolving market. With AI agents leading the way, the future of logistics is faster, smarter, and more customer-focused than ever before.
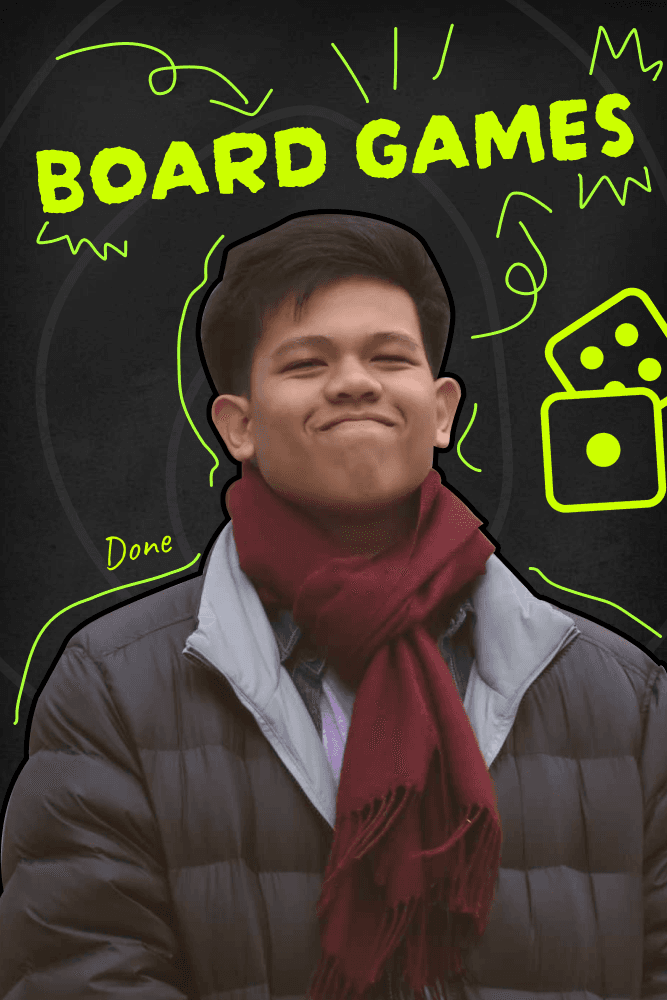
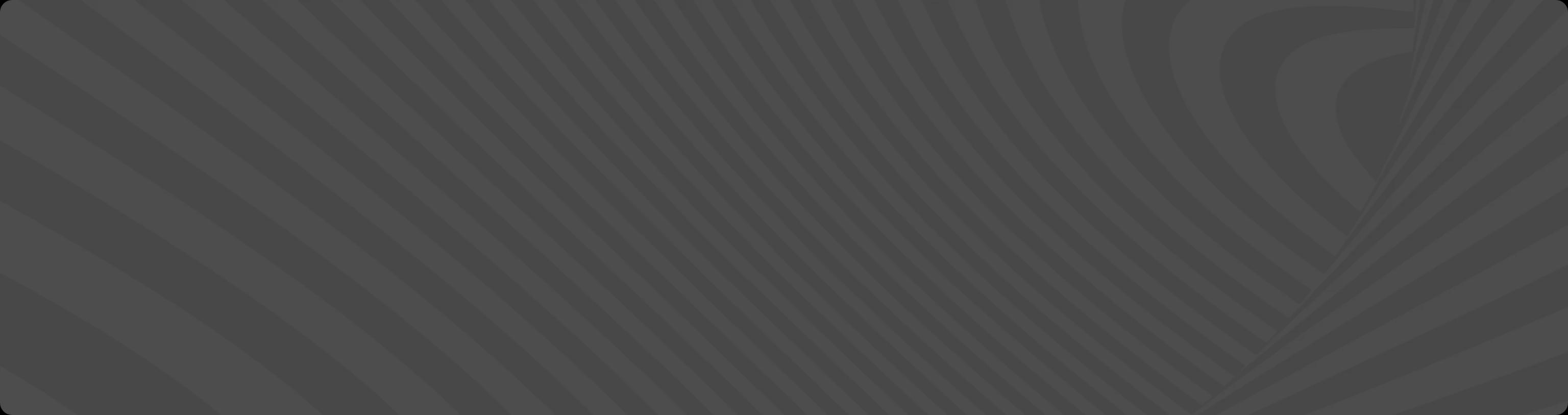
Subscribe to follow product news, latest in technology, solutions, and updates
Other articles for you
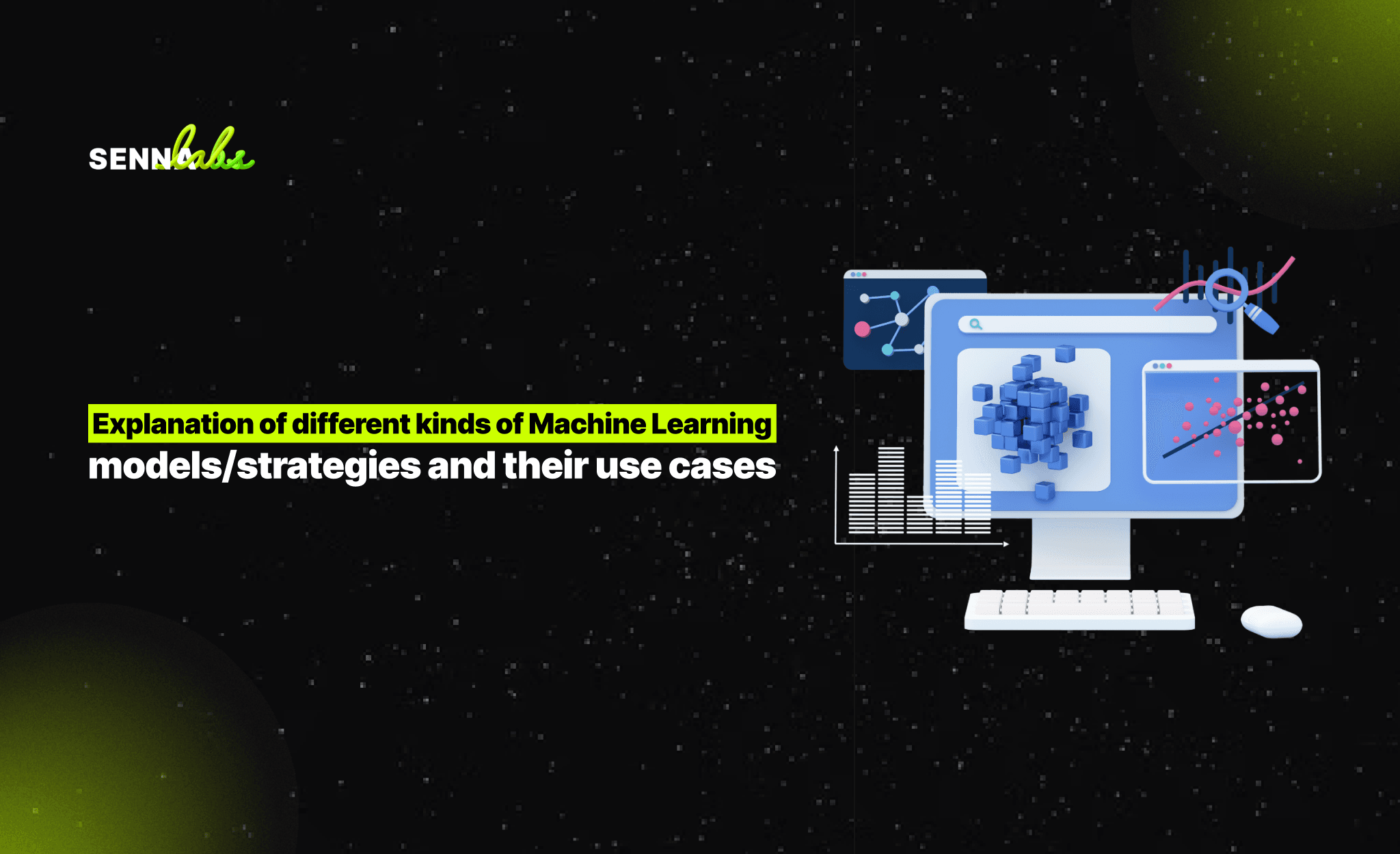
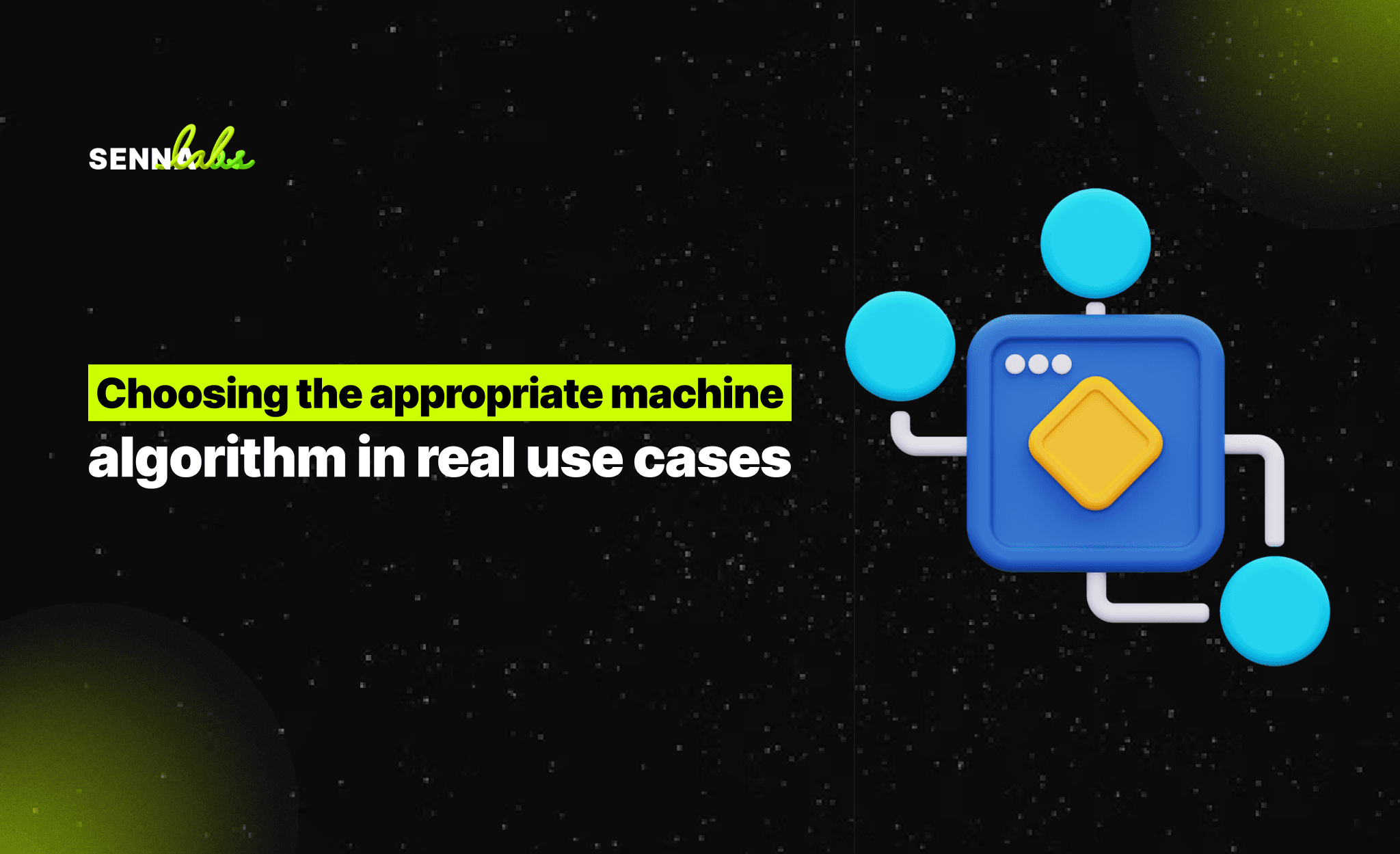
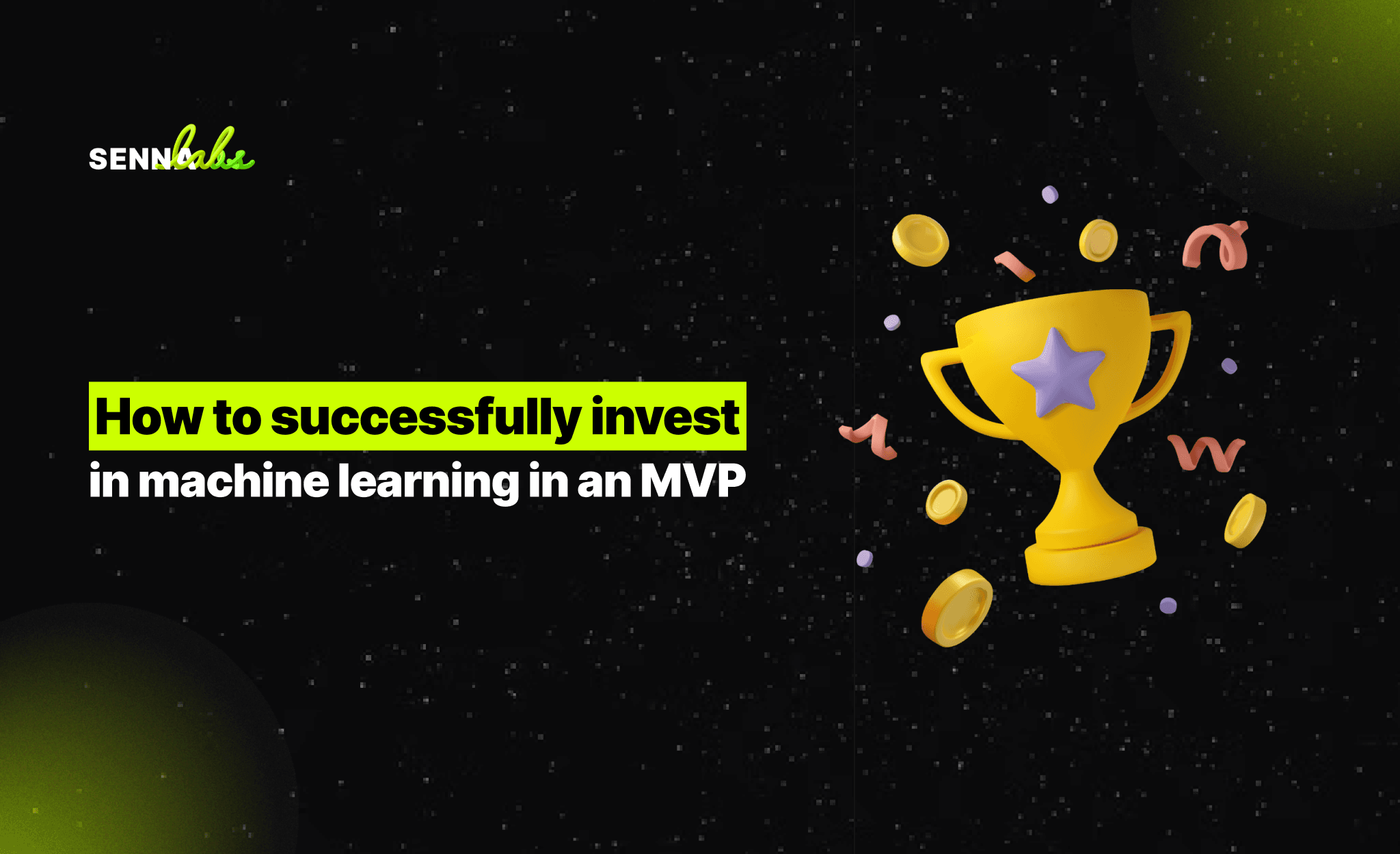
Let’s build digital products that are simply awesome !
We will get back to you within 24 hours!Go to contact us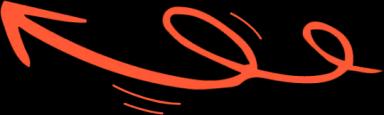
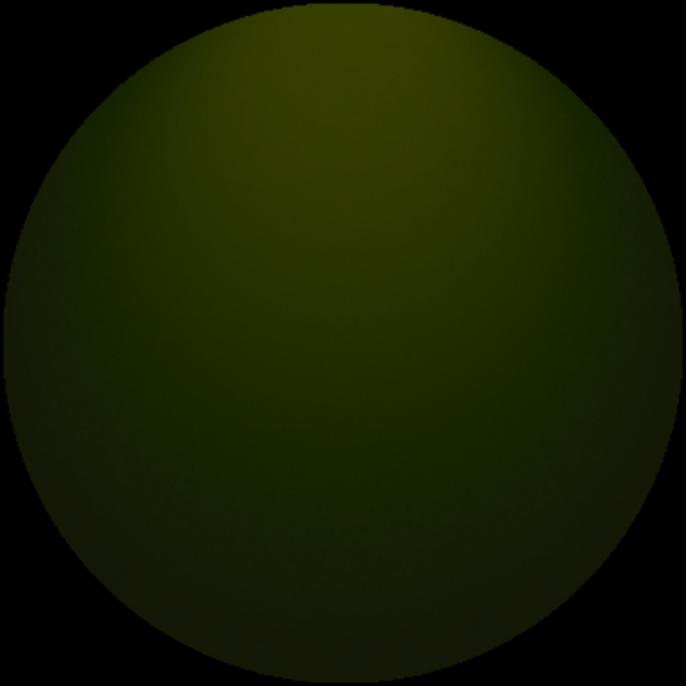
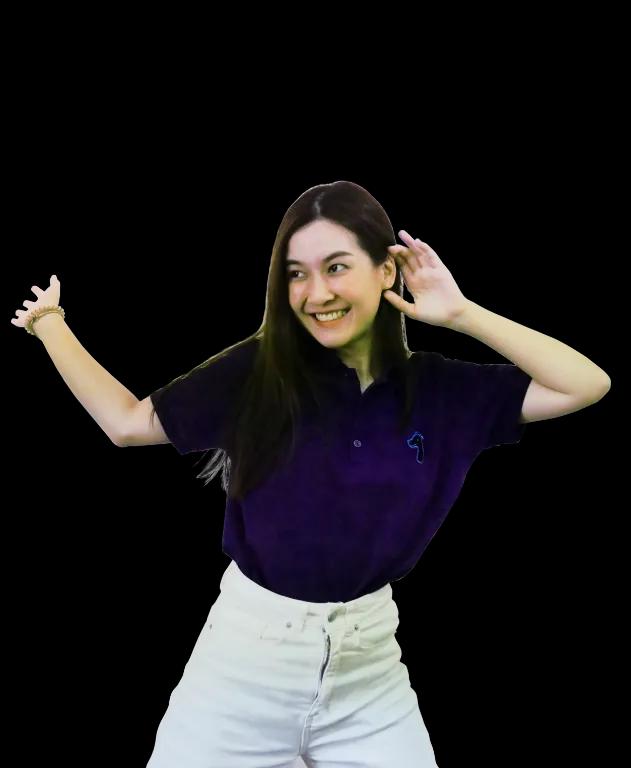
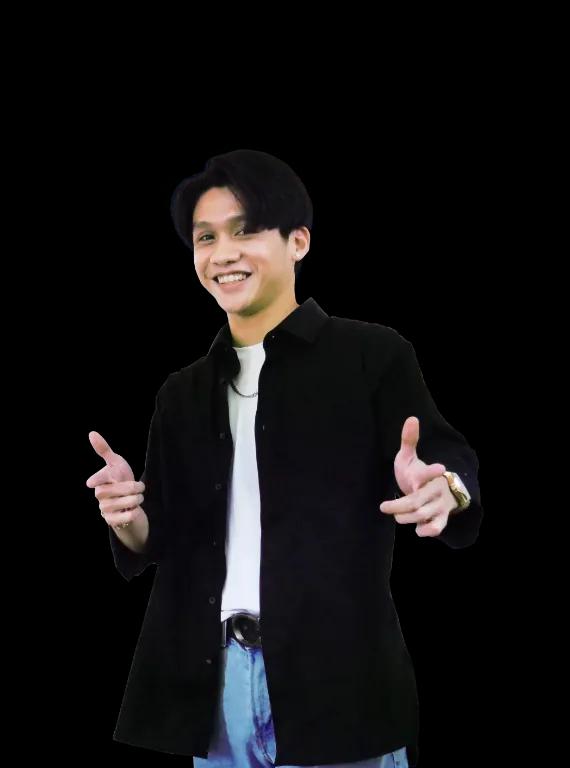
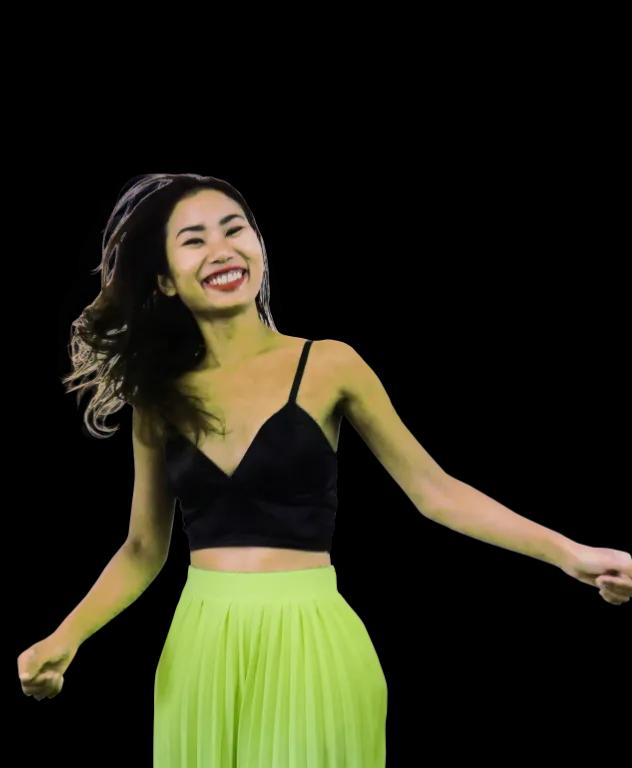
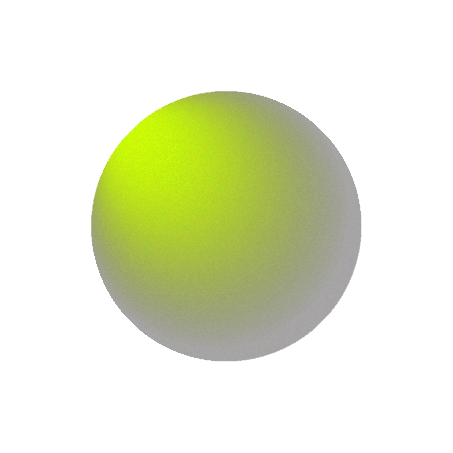
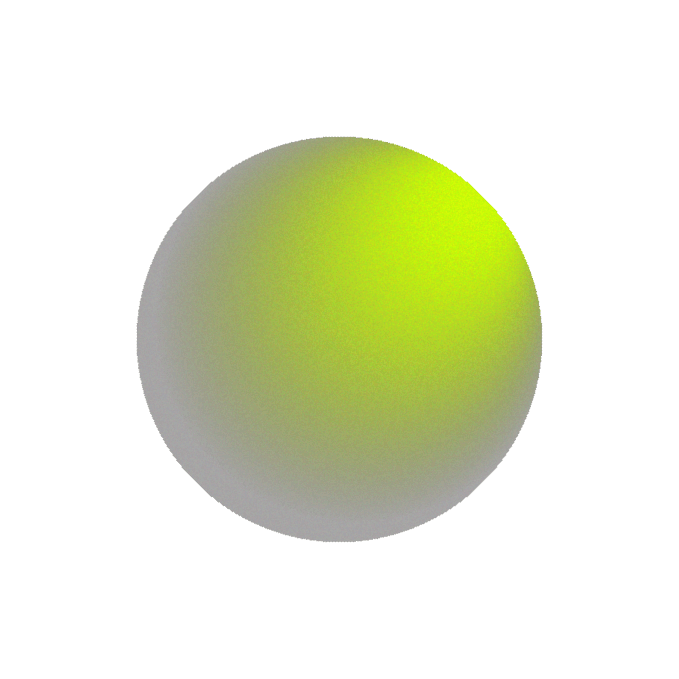
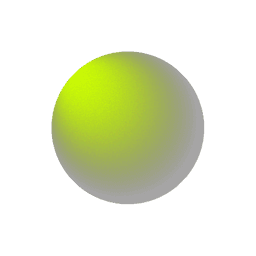
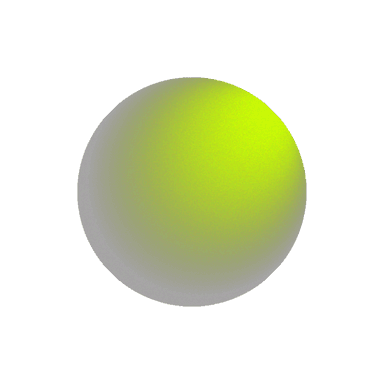
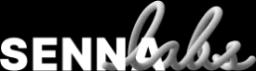